Many concepts such as semantics, intent and topical authority dominate most presentations at SEO conferences today. Search engine performance is evolving at a very rapid pace and if you don’t want to be left behind – you need to keep up with the changes, understand the latest trends and turn this knowledge into effective action.
This article is designed to help you with this.
Thanks to the references to Google patents included here, as well as the analyses of algorithms and language models, you will understand what semantic SEO is.
In addition to the theoretical knowledge, you will also find practical hints and tips to apply to your SEO efforts and writing content tailored to Google’s (and users’) expectations.
Reading this text, you will learn, among other things:
- How search engines have historically changed to arrive at the versions we know today,
- What language models Google uses today to better understand user queries,
- What is semantic SEO and why is it so important for search engine optimisation,
- What user intent is and how does Google seek to satisfy it,
- What are entities and what impact do they have on SEO,
- What topical authority is and how it works,
- What are thematic clusters and how to create them,
- What content hubs are and how to create them,
- How to create content and services that comply with semantic SEO principles,
- What is the future of semantic SEO and how to prepare for it.
What will your knowledge of semantic SEO be useful for?
If you are a:
- SEO specialist: the knowledge contained here will help you to create more effective SEO strategies, especially in the area of information architecture and website content.
- Content marketer / SEO copywriter: knowledge of semantic SEO will shed new light on your work – you will use it to write better (more effective) content.
- Marketer: you will understand how much of a sophisticated tool today’s search engines are and how you can respond to their needs.
If you do not belong to any of the groups mentioned, but SEO is in your area of interest, you will also find issues of interest here.
I also encourage you to watch a webinar on semantic SEO, in which I discuss the key issues surrounding the topic.
Note from the author:
In 2016, I wrote an article about one of the key concepts in semantic SEO, which is topical authority (you can find it here). It has since been read over 100,000 times and is still one of the most popular posts on our blog. Six years in SEO is a lot. Search engines have made huge strides in that time, the SEO industry’s awareness of the issues I describe has also changed dramatically. Therefore, I came to the conclusion that it was a good time to update and expand my previous article with new knowledge and information.
What is semantic SEO?
To understand what semantic SEO is, let’s start by explaining what a semantic search engine is.
A semantic search engine is one that understands the meaning, intention and context of a user’s query. Google has been such a search engine since 2013 or so.
Previously, Google could be spoken of as a lexical search engine that searched by matching queries to documents – without understanding the meaning of those queries. From the user’s point of view, its performance was therefore imperfect.
It is with reference to the semantic way in which a search engine operates that the term semantic SEO was determined. Depending on the source, semantic SEO is defined slightly differently – don’t be surprised if you come across a slightly different definition than the one below:
Semantic SEO is a way of conducting SEO activities that focuses on understanding user intent and aims to fully satisfy that intent. This is done by providing in the content all the answers the user may be looking for, in the form in which they are looking for them. Semantic SEO focuses on a holistic (end-to-end) approach to optimising a website’s content and information architecture.
In the remainder of this article, I will introduce you to concepts, techniques, models and everything related to semantic search and semantic SEO.
How to read an article on semantic SEO?
Please note that the patents presented in the text and how they work do not illustrate the actual performance of the search results.
Google may currently use slightly different techniques than those described in the patents listed – we have no official, fully up-to-date knowledge of how the search engine works. Especially with artificial intelligence developing so rapidly.
Even if any of the patents presented here are not currently in use, this does not mean that they are not worth knowing, as the methodology of operation itself has not changed much.
Google may have changed the language model used for analysis, but it has not changed the way it assesses certain parameters (e.g. it analyses the text differently, but takes the same parameters into account for its assessment).
If you find some parts of this article too difficult to understand due to technical knowledge, skip to the practical tips section. You don’t need to understand all of Google’s patents to achieve good rankings in search results.
History. The road to a semantic search engine
For a full understanding of the issues I will be covering here, it is useful to know the history of changes to the Google search engine. For SEO professionals with a lot of experience, these things will not be new, but if you have been in SEO for less than 10 years, this knowledge may be new to you.
Structured data, i.e. schema.org (2011)
In 2011, most of the major search engines started to use the benefits of artificial intelligence in an attempt to improve the quality of their search results. One important element on this path was the launch of the schema.org dictionary, which was created in 2011 by the major search engines (Google, Bing, Yahoo!). Describing content on the web using structured data has enabled search engines to understand website content much better.
Structured data can be used to describe elements such as media, events, organisations, people, places and much more. Thousands of unique features can now be added to the information on websites.
This standard is used by most major websites today. This provides search engines with a huge database to further develop their algorithms. There will be more about these algorithms later in this article.
Structured data can be described in three different formats:
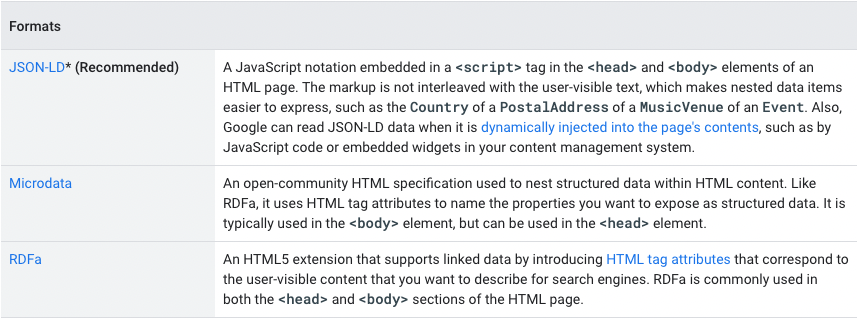
źródło: https://developers.google.com/search/docs/advanced/structured-data/intro-structured-data?hl=en
This is what a sample description in JSON-LD format would look like on a page dedicated to John Lennon:
{ "@context": "https://json-ld.org/contexts/person.jsonld", "@id": "http://dbpedia.org/resource/John_Lennon", "name": "John Lennon", "born": "1940-10-09", "spouse": "http://dbpedia.org/resource/Cynthia_Lennon" }
We can come across the schema.org standard directly in the search results.
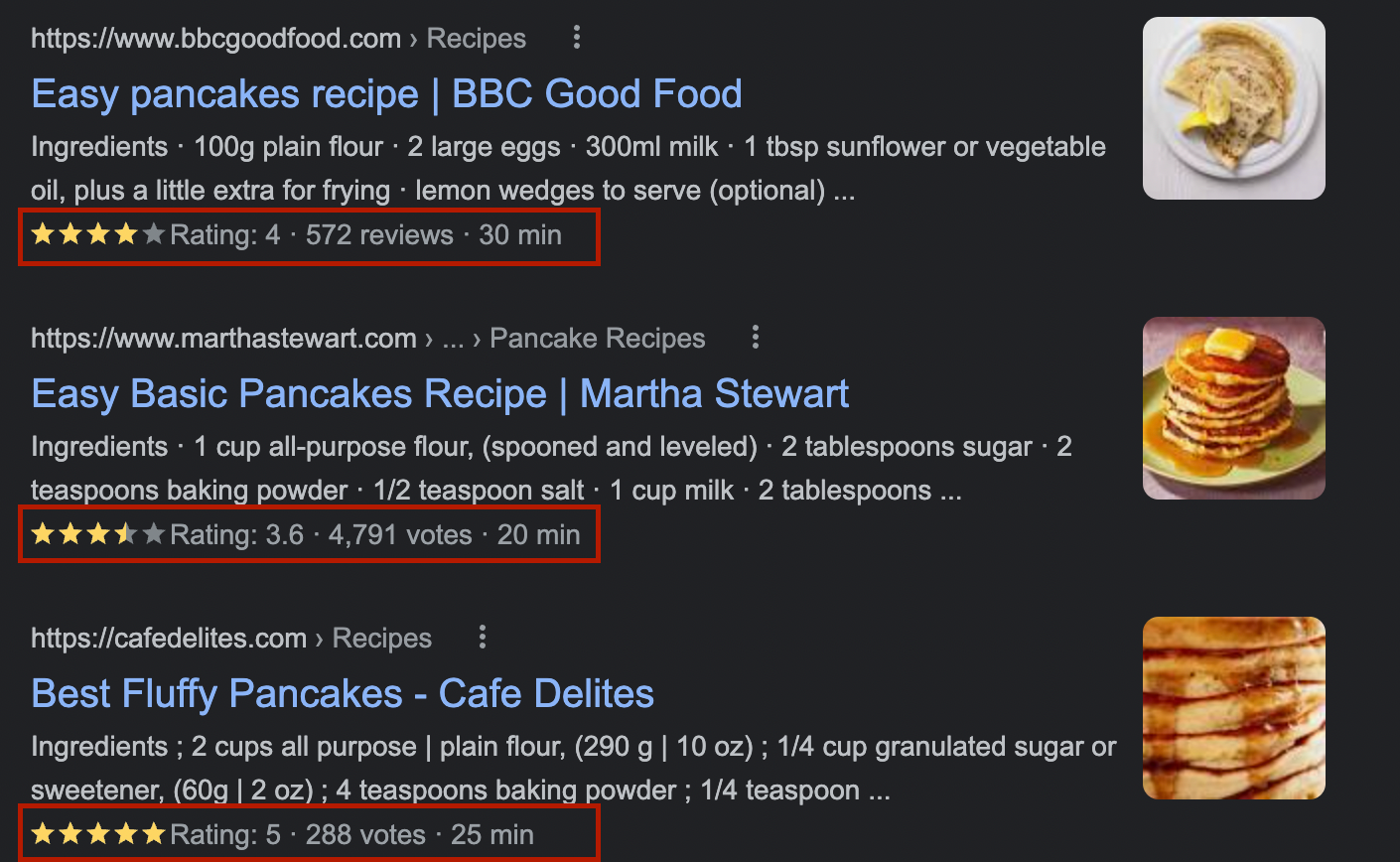
The items highlighted in the screenshot (user ratings) are extended results, for which the data is derived from structured data described by the Schema standard.
Using a structured data testing tool, it is possible to test exactly which microformats a site uses.
The key here is the word ‘structured’ – search engines, thanks to the schema.org dictionary, started to receive data in the form of the algorithms expected (the search engine algorithm did not have to ‘guess’ that a page was, for example, a recipe – it got the data described). In 2011, the algorithms could not quite cope with unstructured data; without schema, the creation of a semantic search engine would not have been possible at that time.
Describing the information using the schema.org standard does not affect the position in the search results, but can have a significant impact on the CTR (click-to-impress ratio). Simply describing information using structured data also does not guarantee that it will appear in search results.
Additional sources of knowledge:
- https://developers.google.com/search/docs/advanced/structured-data/intro-structured-data?hl=en
- https://json-ld.org/
- https://schema.org/
- https://rdfa.info/
- https://html.spec.whatwg.org/multipage/
Things not strings – knowledge graph (rok 2012)
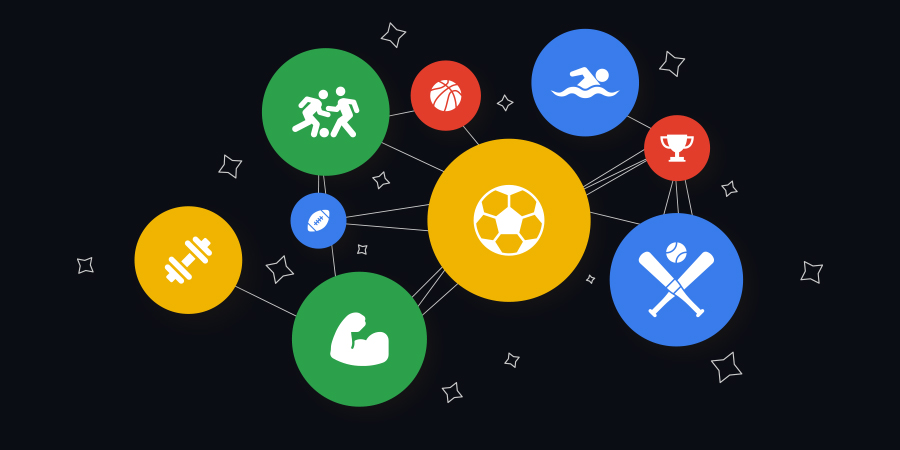
Until 2012, Google’s algorithms did not understand queries. The query ‘palace of culture’ for the algorithm was two words. It did not understand their meaning. It tried to match the best documents from the internet based on known parameters: metadata optimisation, content or links.
In May 2012, Google announced the introduction of the knowledge graph, titling the change: “things, not strings”. Among other things, thanks to data collected from structured data (the data also came from Wikipedia or the CIA World Factbook), the search engine’s algorithm began to understand the meaning of words. “Palace of culture” ceased to be a conglomeration of two words, it started to be a place.
As of 2012, Google was able to recognise 500 million places and 3.5 billion facts (today this figure is certainly much higher).
A video in which Google presents a knowledge graph:
For users, the introduction of the knowledge graph has had the effect of changing the appearance of search results. When searching for places, people or other information that is stored in the knowledge graph, Google displays additional information.
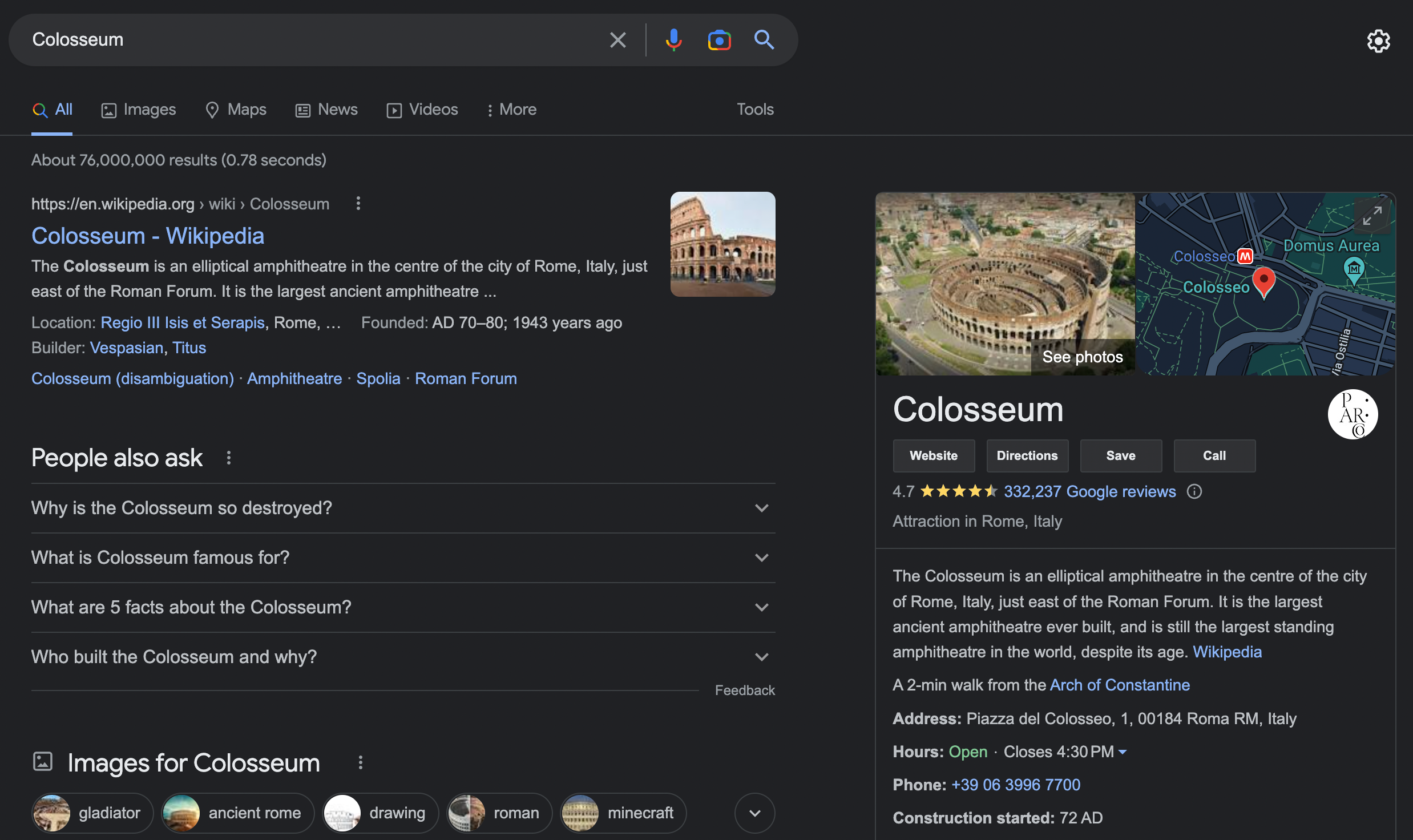
For SEO specialists, this was not a positive development.
For queries where the knowledge graph appeared, the CTR dropped dramatically. For example, for the query ‘how old is Tom Cruise’, the number of click-throughs from Google dropped to practically zero as Google started to provide answers directly in the search results.
Today, the knowledge graph can be found on 10-12% of desktop results and 15-20% of mobile results (depending on the market).
The introduction of the knowledge graph did not just change the appearance of search results, but also Google’s ability to understand content and user intent. This change became more apparent gradually over the following years.
Hummingbird algorithm (2013)
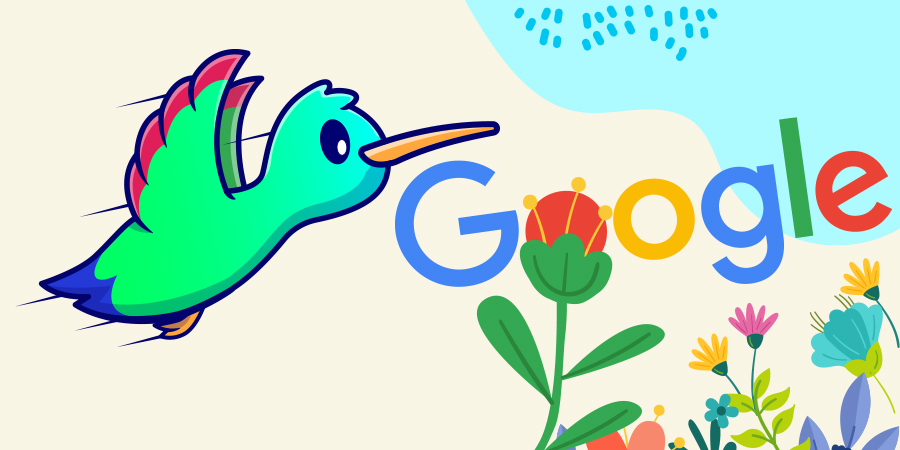
During the 2011-2016 period, when Google was making successive major changes to the algorithm (symbolised by various animals), the SEO industry felt no small amount of fear. Algorithms such as Panda and Penguin were wreaking havoc on search results (they used to be updates!).
However, this was not the case with the Hummingbird algorithm.
The update passed rather without much of an echo. It did not change search results overnight. Unlike algorithm updates such as Panda and Penguin, which acted as an add-on to the main algorithm (Penguin version 4.0 was introduced into the main algorithm), the Hummingbird update was a change to the search engine’s main algorithm from the start (it became part of its operation).
Google described this as the biggest algorithm change since 2001. Matt Cutts noted in an interview that the update affected 90% of search results.
We regard the Hummingbird update as the beginning of semantic SEO.
These are the beginnings of natural language processing (NLP) algorithms in the search engine. Search engine users at that time were already more aware, typing longer and longer queries that resembled natural language. The search engine mechanism had to adapt to this change.
Until the introduction of Hummingbird, typing in the query ‘Chinese food’ would probably direct to recipe pages. After the introduction of Hummingbird, the user would receive a list of the best restaurants in the area.
The algorithm itself has made the biggest changes in local queries. Since its introduction, more and more results in addition to the standard website links have included the so-called ‘local pack’, i.e. results from Google My Business.
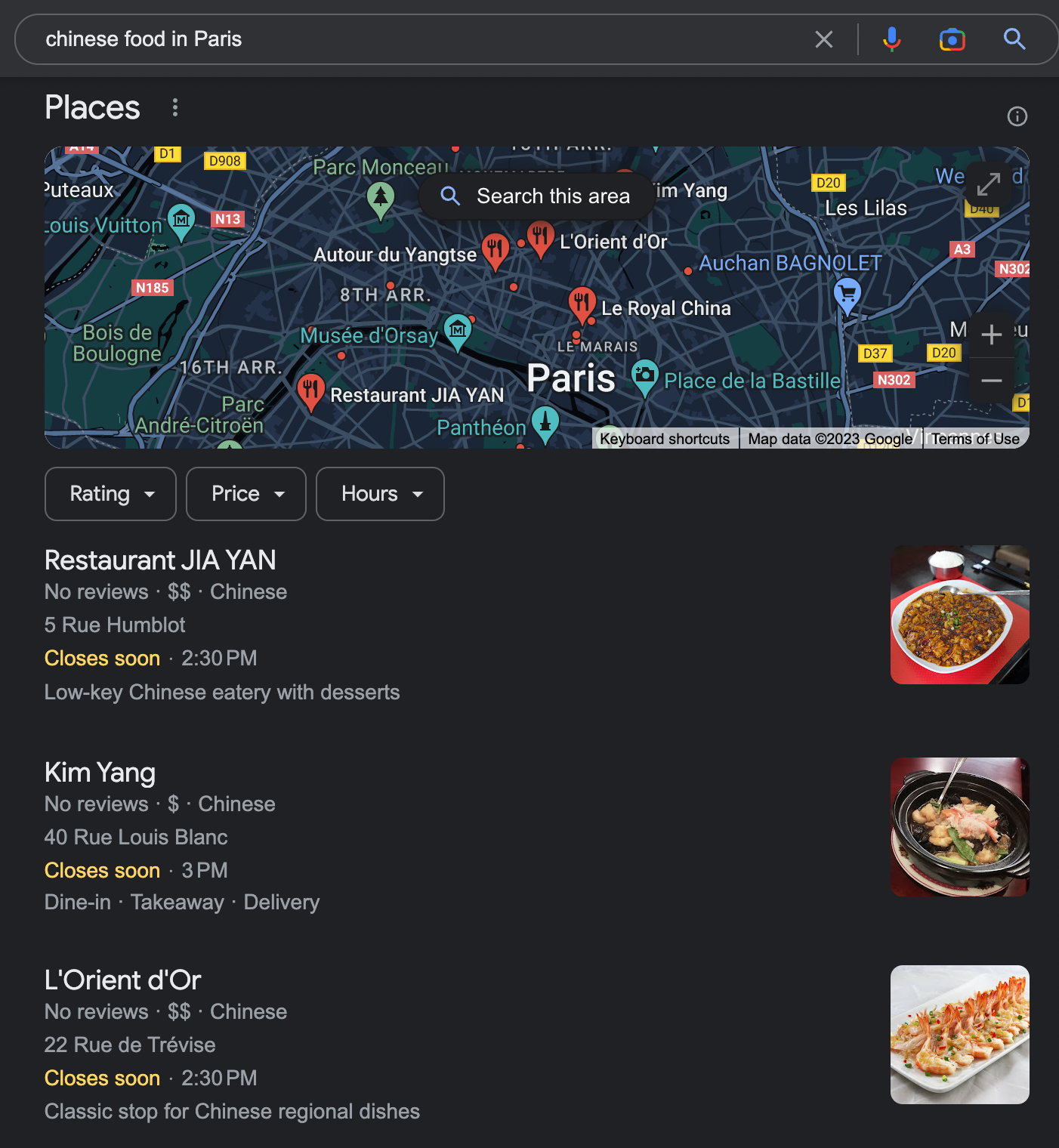
Currently, depending on device and location, up to around 45% of all results contain local results.
2013 was also the year that voice search started to gain popularity. Google needed to understand natural language to respond to this need.
Today, Google uses much more advanced NLP models (I describe them later in the article). 10 years in a technology where development is exponential is ages.
Panda 4.0 (year 2014)
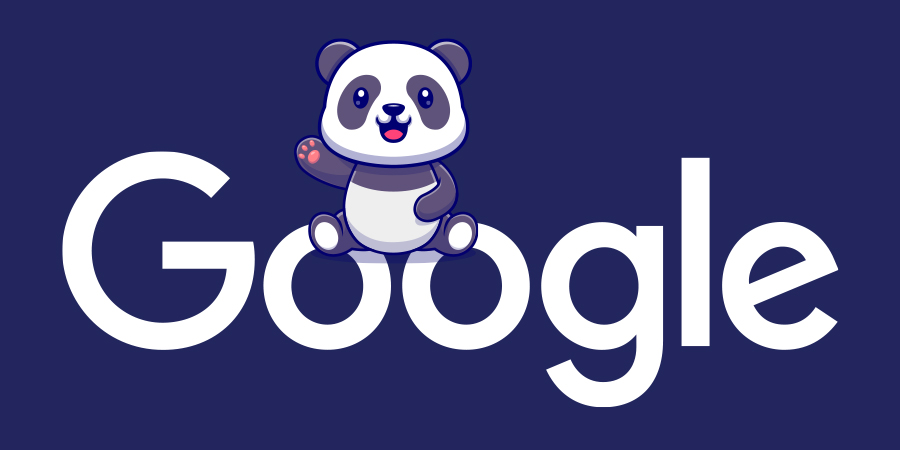
There have been several updates to the Panda algorithm (the first in 2011). Some concerned copied content, others thin content – thanks to Panda, today every SEO specialist knows that Google does not like copied and irrelevant content.
Panda 4.0 introduced the concept of topical authority to SEO (I will explain it in detail later), i.e. rewarding sites that are expert in a particular field.
The first reports on Panda 4.0 were that it affected 7.5% of all search results. Google itself claimed that this update, like previous iterations of Panda, was designed to combat spam. Google has never confirmed that this update started to give a premium to domain expert sites, but this is what all the tests and expert predictions point to.
A great study on this topic was conducted by Razvan Gavrilas of Cognitive SEO.
During the period of the introduction of the fourth version of Panda, small sites that were optimised for a limited number of keywords and were not expert in their fields began to disappear from the search results. The results began to be dominated by large or specialised sites on specific topics.
How does Panda 4.0 work?
Before the Panda 4.0 period, different domains in the results for the two phrases below would not have been surprising:
- Type 1 diabetes
- Type 2 diabetes
After the introduction of Panda 4.0, the results for such two phrases will be dominated by similar domains, as both phrases belong to the same field of knowledge.
In 2014, Google already had a knowledge graph and the first algorithms to study intent. It was also examining topical authority. The search engine thus already had all the ingredients of what we today call semantic SEO. The following years saw an evolution in these areas and the introduction of more and more advanced solutions.
RankBrain (2015)
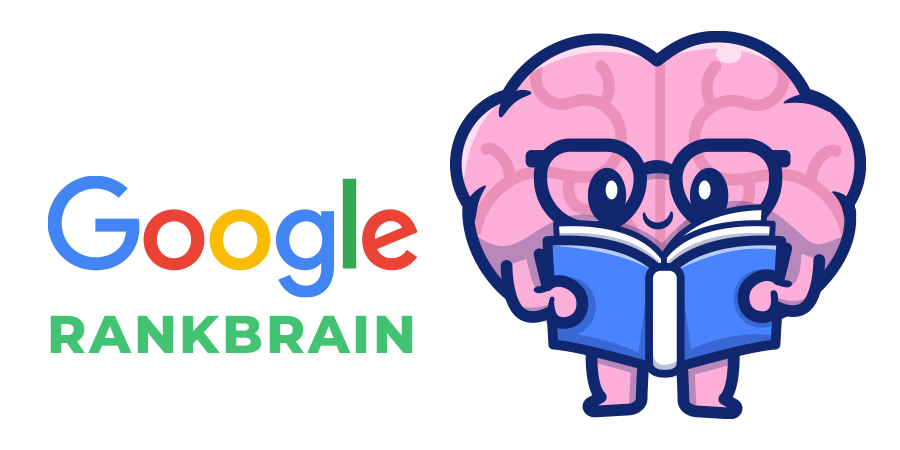
RankBrain has created quite a stir in the SEO industry. It can hardly be otherwise when one of the most important engineers at Google, Paul Haahr, at the SMX conference (in 2016) says that they themselves do not fully understand how RankBrain works.
RankBrain is the first manifestation of unsupervised artificial intelligence in search results. And it is a self-learning artificial intelligence, as Paul Haahr’s words indicate.
Until the introduction of RankBrain, all algorithm rules were created by Google engineers – after that, artificial intelligence was able to manage the rules (rules are not predefined). According to research, RankBrain in 2015 predicted results 10 p.p. better than engineers.
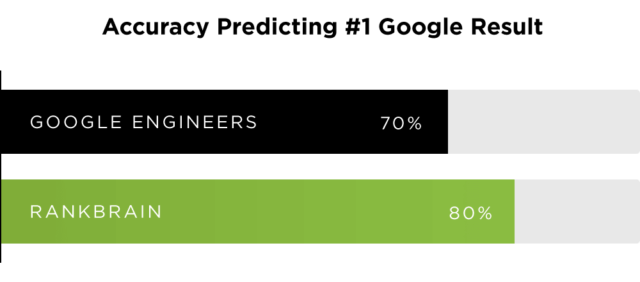
RankBrain performs several tasks:
- Understanding new, never-before-entered queries (according to available research, such queries account for 15% of all queries)
- Understanding long queries (e.g. those from voice search)
- Better linking of concepts to pages
- Analysis of the user’s historical behaviour to determine his/her preference for the results obtained
How does RankBrain affect search results?
An example of how RankBrain works can be seen, for example, with the query “black console made by Sony”. – thanks to RankBrain, Google knows that the query is about PlayStation.
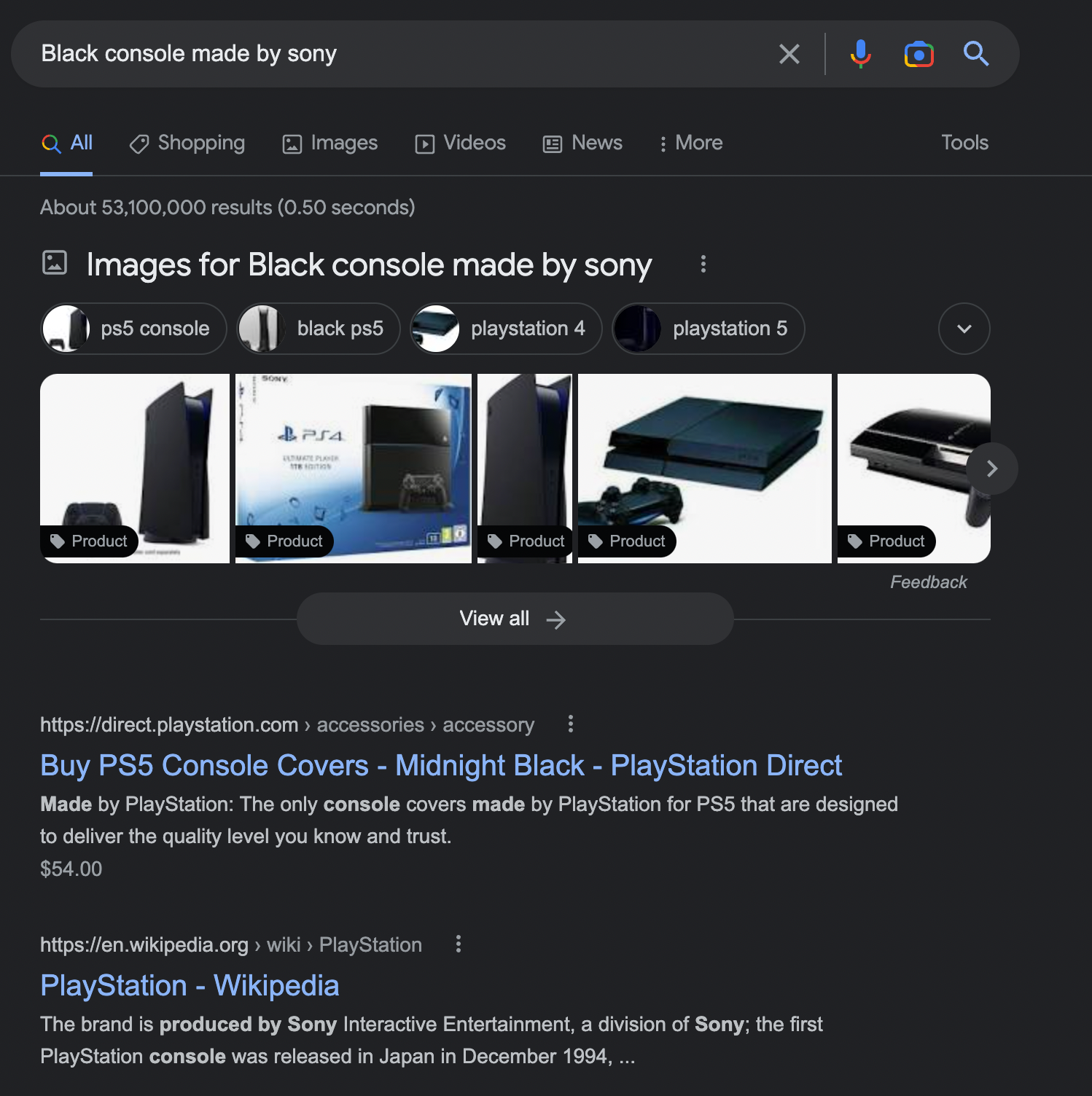
An example of how RankBrain works can be seen, for example, with the query “black console made by Sony”. – thanks to RankBrain, Google knows that the query is about PlayStation.
The fact that the search engine has been using terms rather than phrases for some time (thanks partly to RankBrain) has forced a slightly different approach to keyword research.
If two phrases are very similar and pursue the same intention, Google will combine them into one term. This makes it possible to appear in the search results even for phrases that do not appear in the content, and one subpage can be visible for thousands of keywords.
Example: “Pregnancy and due date calculator” on medonet.pl
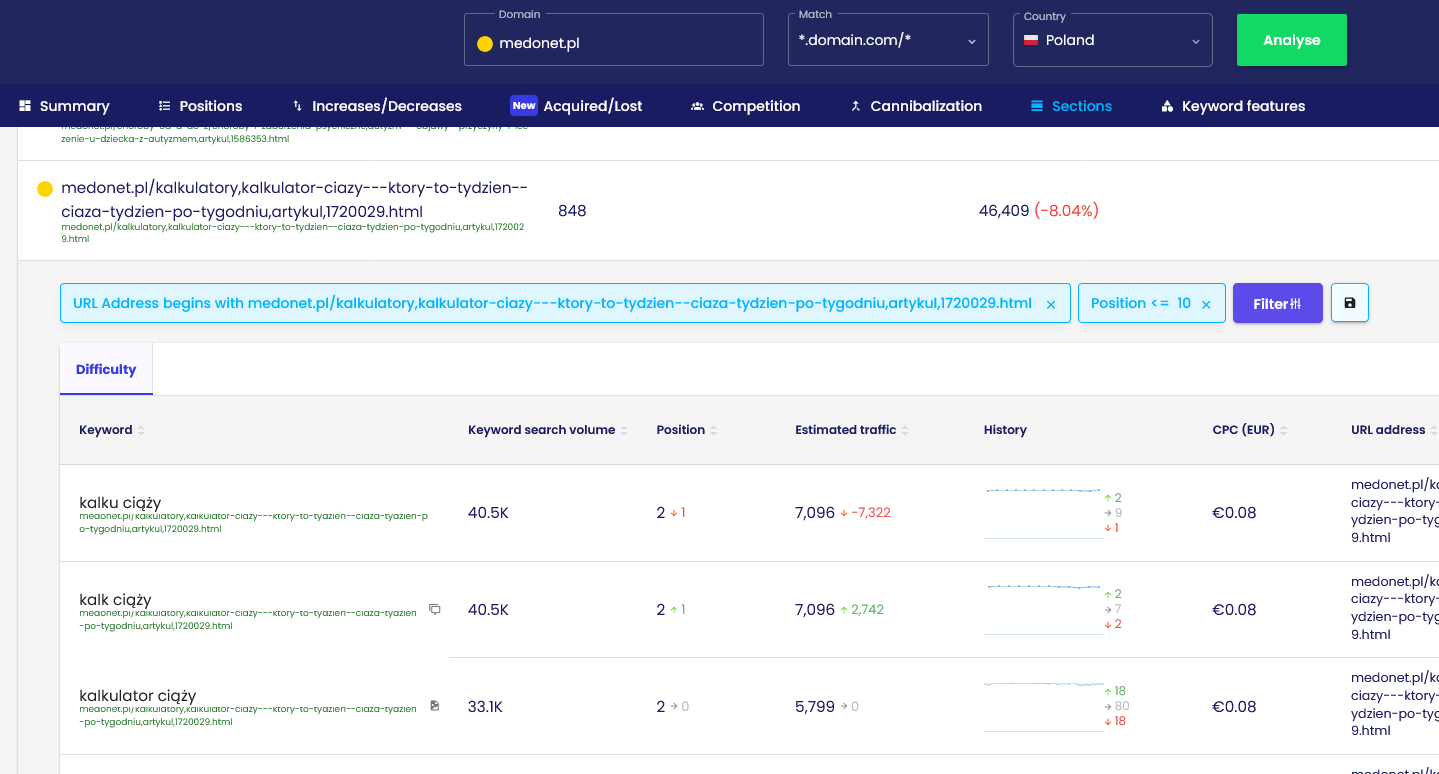
This article is visible on as many as 856 keywords in the TOP10, and not only for phrases such as “pregnancy calculator”, but also:
- How many days is the pregnancy – 1st position
- What week of pregnancy am I – 2nd position
- date of birth – 6th position
None of these phrases appear in the content in a literal sense. There are dozens of examples of such phrases in this article alone.
Does RankBrain take behavioural factors into account?
There is a lot of text on the internet about RankBrain using behavioural factors to determine rankings, such as CTR or dwell time. This was the original prediction of the industry.
However, there was a 2019 AMA on Reddit with Google’s Gary Illyes, in which he answered questions about RankBrain, among other things. Gary provided some interesting information that threw a big question mark into the issue of RankBrain’s use of behavioural factors:
“RankBrain is a PR-sexy machine learning ranking component that uses historical search data to predict what would a user most likely click on for a previously unseen query.”
“Dwell time, CTR, whatever Fishkin’s new theory is, those are generally made up crap. Search is much more simple than people think.”
Of course, as an SEO industry, we know that Google repeatedly makes vague statements about how its own mechanisms work. There are now both a number of tests confirming the claim that behavioural factors do indeed matter, and tests refuting this claim.
Google here emphasises that behavioural factors do matter, but within the search results, not the page itself. Even if the thesis of some SEO experts is true, this is not necessarily the effect of RankBrain.
Neural matching (2018)
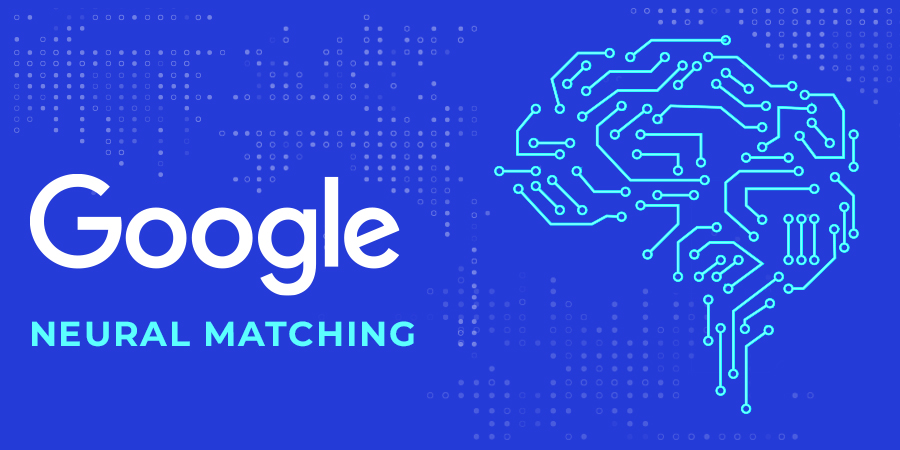
Neural matching is a type of neural network that functions within Google’s algorithm.
In practice, Neural matching, using artificial intelligence (neural networks), allows the search engine to understand the query better <> page relationship, especially for queries that do not have an explicit intention.
These relationships are a key element for a semantic search engine to work correctly – and for effective semantic SEO efforts.
Google refers to Neural matching as a super dictionary of synonyms. The introduction of this element is another step towards better matching results to user intent.
From a tweet by Google’s Danny Sullivan, we can learn that at that time (2018), Neural matching influenced almost 30% of all queries. For many SEO specialists, the familiar algorithm is the TF * IDF algorithm – Neural matching is a much more advanced way of solving a similar problem.
How does neural matching work?
According to a Google research paper, Neural Matching matches results based solely on the query and content of the site, leaving out all other algorithmic factors. When this algorithm was introduced, there was a big fear in the SEO industry that Google would stop taking factors such as links into account.
These concerns proved unfounded, as it acts as a supplement to the main algorithm and only in the area of pages that are already high in the search results.
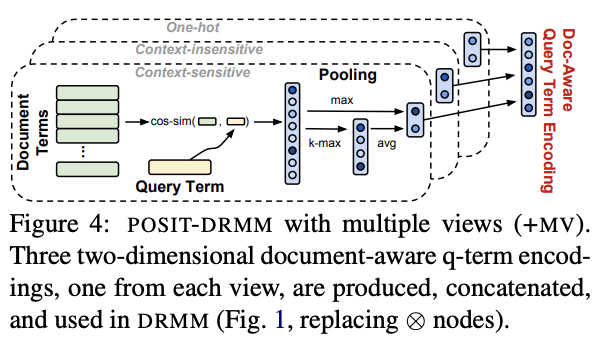
A diagram of how neural matching works.
An example of how Neural matching works can be seen from the following query:
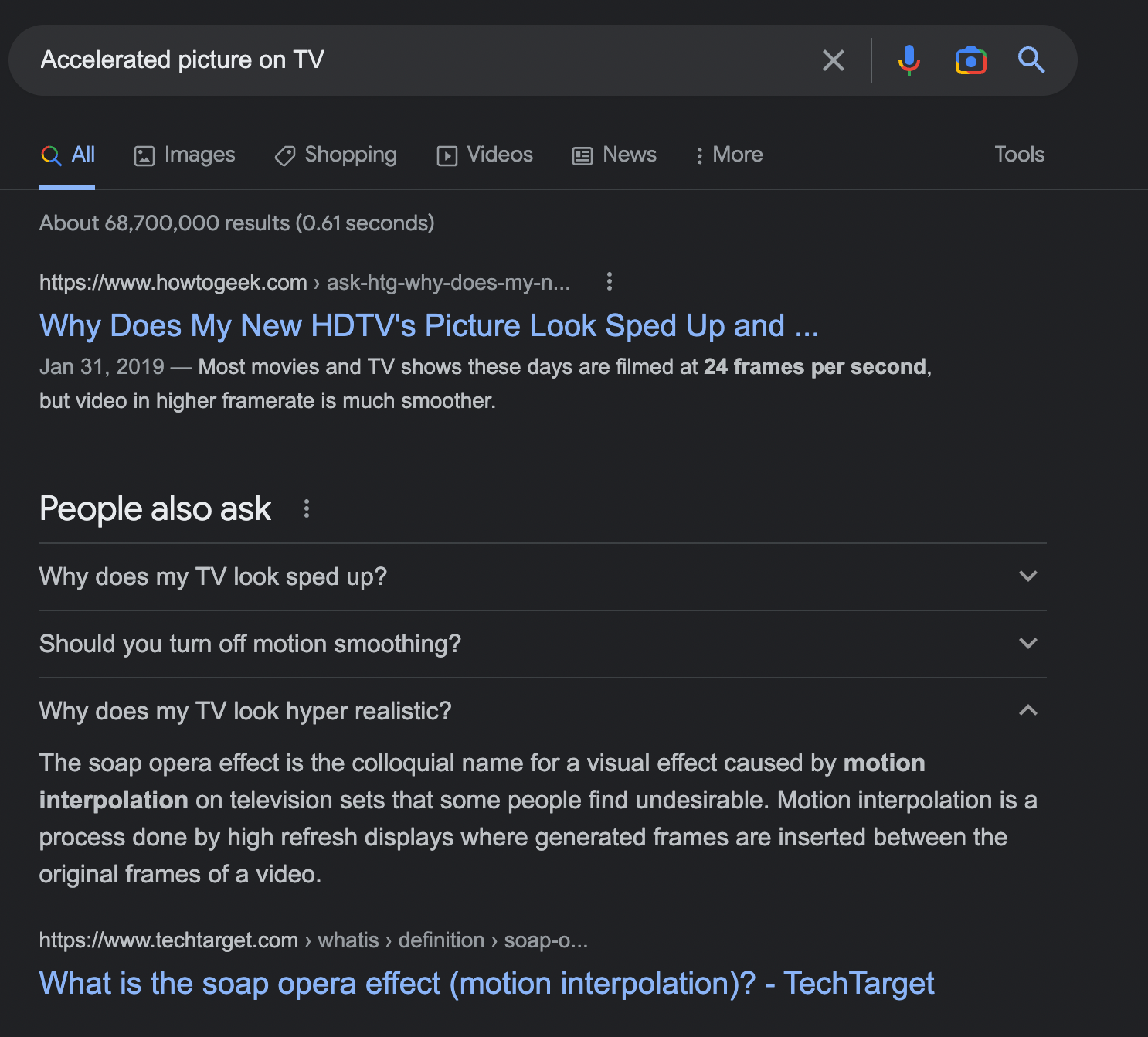
The accelerated image on the TV is called the ‘soap opera effect’. Google is able to detect this link by linking the user’s query to the entire document (page).
As a result, in extreme cases, pages may appear in search results that do not contain the words in the query.
It may seem that Neural matching works on the same principle as RankBrain. However, there is a difference between these algorithms and Google explains it this way:
- RankBrain allows the search engine to link terms to pages (e.g. PlayStation)
- Neural matching allows the search engine to link words to concepts (soap opera example)
The differences between Neural matching and RankBrain, Google explained in one of a series of tweets in 2019.
You can read more about Neural matching on the Google blog.
BERT (2019)
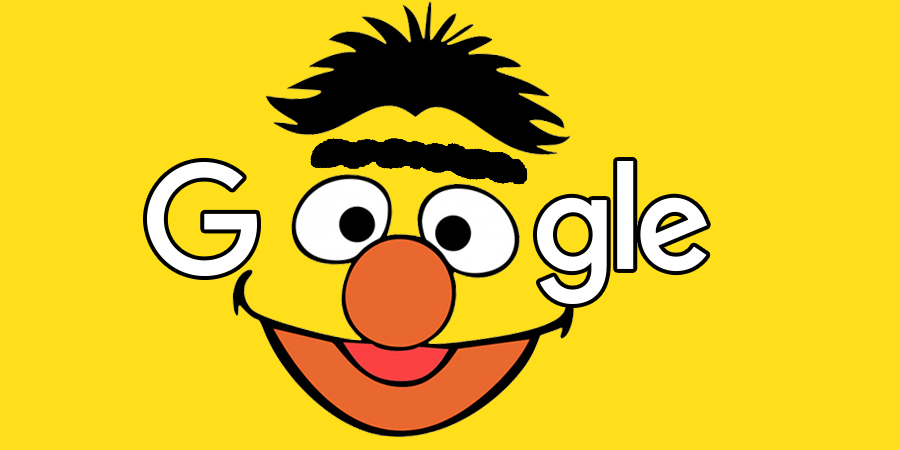
In October 2019, Google took another step towards better understanding queries and user intent.
BERT (Bidirectional Encoder Representations from Transformers) is a language model with ‘Bidirectional’ in its name, which is quite significant. For it means that BERT allows us to understand the meaning of words in relation to the words that come before and after the word.
BERT is a self-learning algorithm. It can learn on one language and apply this knowledge in another. BERT can be trained for different types of tasks. In machine learning, the term fine-tuning is used to refer to training a model to fulfil other tasks – and this is what applies to BERT.
About a model that did not understand the context
Previous methods of determining semantic links, such as the Word2Vec model, have been context-free.
Word2Vec creates single word vector representations. For example, the word ‘woman’ will have the same representation in this model in the query ‘pregnant woman’ as ‘sleeping woman’.
As a result of this action, algorithms of this type are not able to analyse the context of the query.
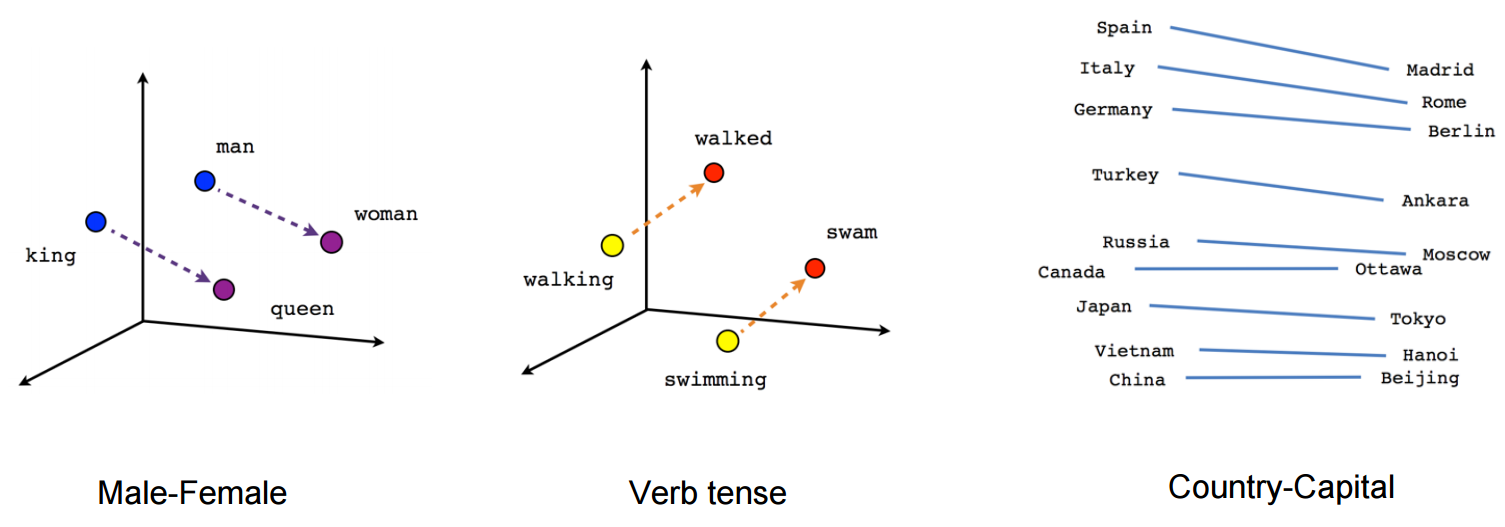
Scheme of operation Word2Vec. Źródło: https://israelg99.github.io/2017-03-23-Word2Vec-Explained/
The example above shows that by converting words into vectors, the Word2Vec model can determine related words. However, these are devoid of context.
If you want to see how the Word2Vec model works, watch the video below:
You can check the operation of the Word2Vec model on this page.
How does BERT work?
Word2Vec is a great way to determine semantically related words. However, in search engine queries, every word is important; any word can change the meaning of the query. This gap is filled by BERT.
An example of how BERT works in search results, for the query ‘2019 does a Brazilian traveller need a visa to the US’:
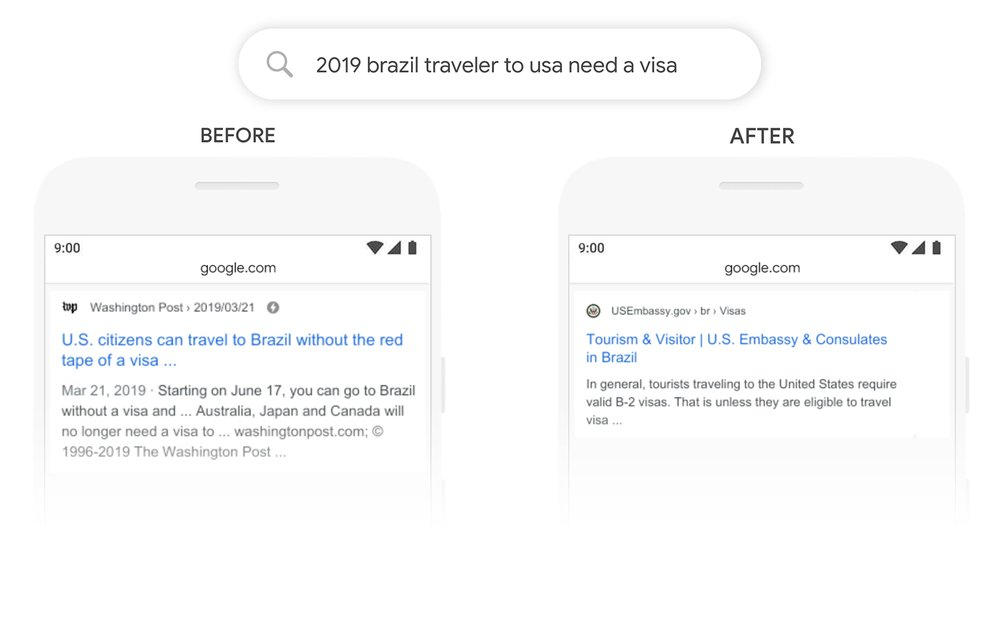
source: https://blog.google/products/search/search-language-understanding-bert/
Prior to the introduction of BERT, Google did not understand the relationship Brazil > USA (the word to is key here) and also returned information for travellers from the USA to Brazil in the results, which was inconsistent with the intent of the query.
BERT is also trained to understand relationships between sentences.

It can recognise whether two random sentences are consecutive sentences.
The effectiveness of BERT
SQuAD2.0 is a project with a database of more than 100,000 questions that contain the answer and those that cannot be answered. The effectiveness of the models is measured by the effectiveness of the answers to the questions in the mentioned database.
BERT was launched in 2019 – and, as you can see from the list below, there are models available today that perform better (note that this test only tests one type of task!).
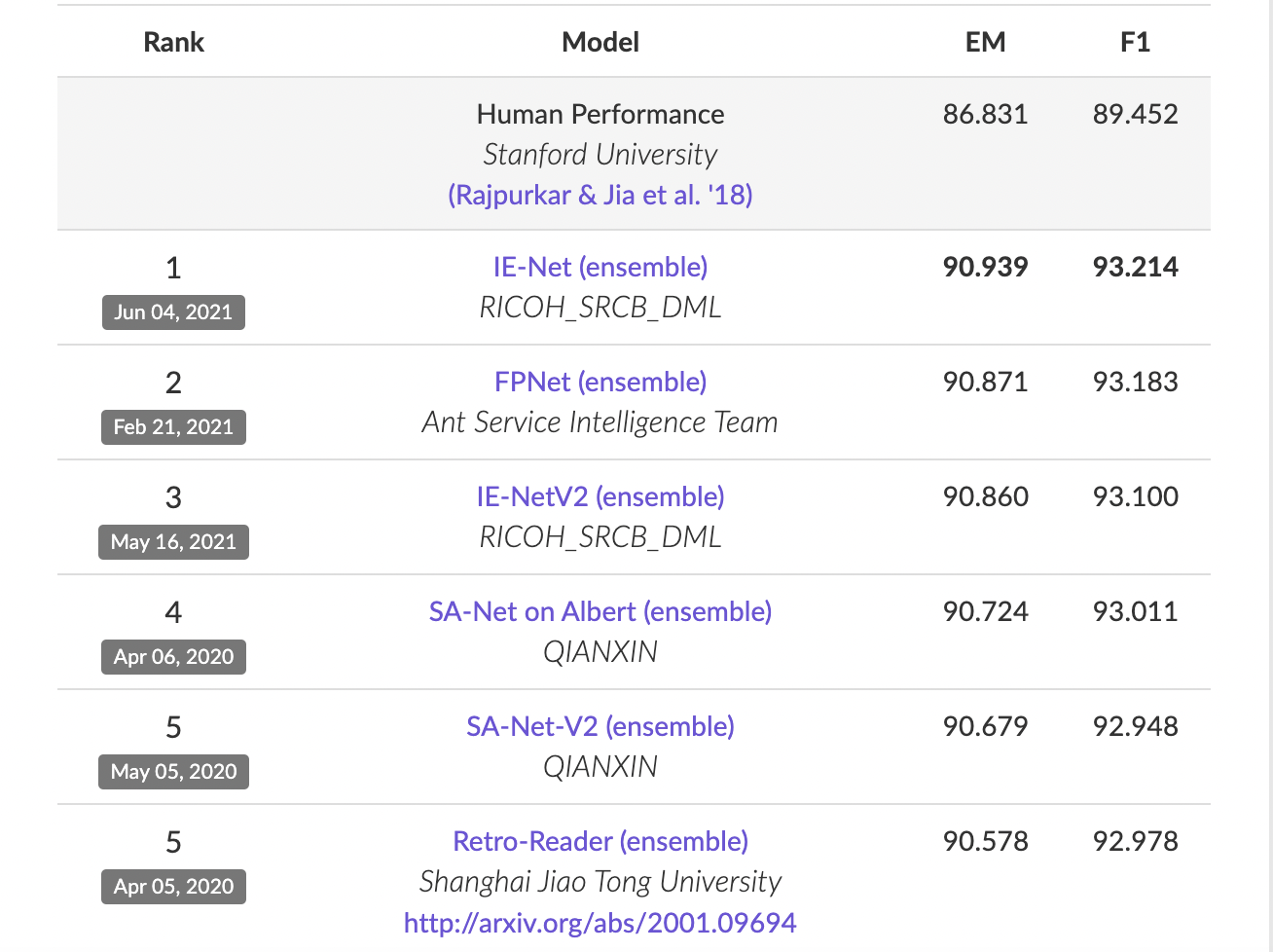
Top models from the study indicated by Google. BERT achieved a score of EM = 87.4, F1 = 93.16. Source: https://rajpurkar.github.io/SQuAD-explorer/
What tasks can BERT be trained for?
As I mentioned, BERT is a language model that can be trained for various tasks. Google in its research paper points out some of these:
- Sentiment analysis (e.g. whether a statement on the internet is positive or negative)
- Question answering system
- Predicting the next part of a sentence
- Determining the most important words in a text
A lot of complicated statements have been made here, but if you think that using models like BERT is difficult, I want to lead you astray. There are plenty of trained models on the internet, and with basic Python coding skills you can enjoy the benefits of this language model.
- Google promises that anyone can train their own BERT model in about 30-60 minutes . Allegro, among others, uses its own BERT model and has made it available for free for further use.
- BERT is an open source model, you can use it for free.
- You can also check out BERT‘s other trained models in Polish.
- You can read more about BERT in a document from Google.
Concepts in semantic SEO
In semantic SEO, there are a set of concepts that often come up. In this section I will try to introduce them. They are used in a variety of contexts, so my explanation may not apply to every context in which you will hear the term. Some people also use these terms in different senses. Don’t get attached to them – it’s important to understand their essence and impact on SEO.
User intent
If you have read the previous part of this article, you will know that Google places extraordinary importance on responding appropriately to user intent. This is written into its mission statement and its actions in recent years prove that this is no mere platitude.
To get a good understanding of how Google views intent it is worth looking at the Search Quality Evaluator Guidelines. This is a document for the Search Quality Evaluator team (Google has one in Ireland, its purpose is to evaluate pages in search results and support the work of the algorithms). The whole document is available here (starting on page 74).
What is user intent?
Google defines it this way:
“When a user types or dictates a query they are trying to achieve a goal. We refer to this goal as intention.”
In Chapter 12 (12.7), Google talks about what intentions should be highlighted:
- Know or know simple: In thecase of the know intention, the search engine user wants to expand his/her knowledge on a certain topic (e.g. by typing Madonna). In the case of the know simple intention, the user is interested in simple information on a topic or fact (e.g. how old is Madonna).
- To: theuser has the intention “I want to do something”, e.g. download a game, buy something. He types in, for example, “what is my bmi?” wanting to take a bmi test. Google also distinguishes here the intention “device action” – when the user wants the phone to perform an action for him, he types or says e.g. “navigate to the palace of culture”.
- Website: or navigation intention. A user with this intention wants to visit a particular website. He types in, for example, “facebook”.
- Visit-in-person: a user with this intention wants to visit a place in person. He types or says ‘Chinese restaurants’.
How does Google determine user intent?
Many of the NLP and AI models I mentioned earlier are used throughout the process (Hummingbird, RankBrain and BERT algorithms were key here). Google additionally uses:
- User language and location
- Demographic profile (you do not need to be logged in; Google determines your demographic profile based on your previous queries – you can checkit yourself)
- Information from previous searches (RankBrain)
- Behavioural factors (we are talking about behavioural factors in search results, not interaction with the website)
In practice, depending on the location or language, the same query may have a different intention.
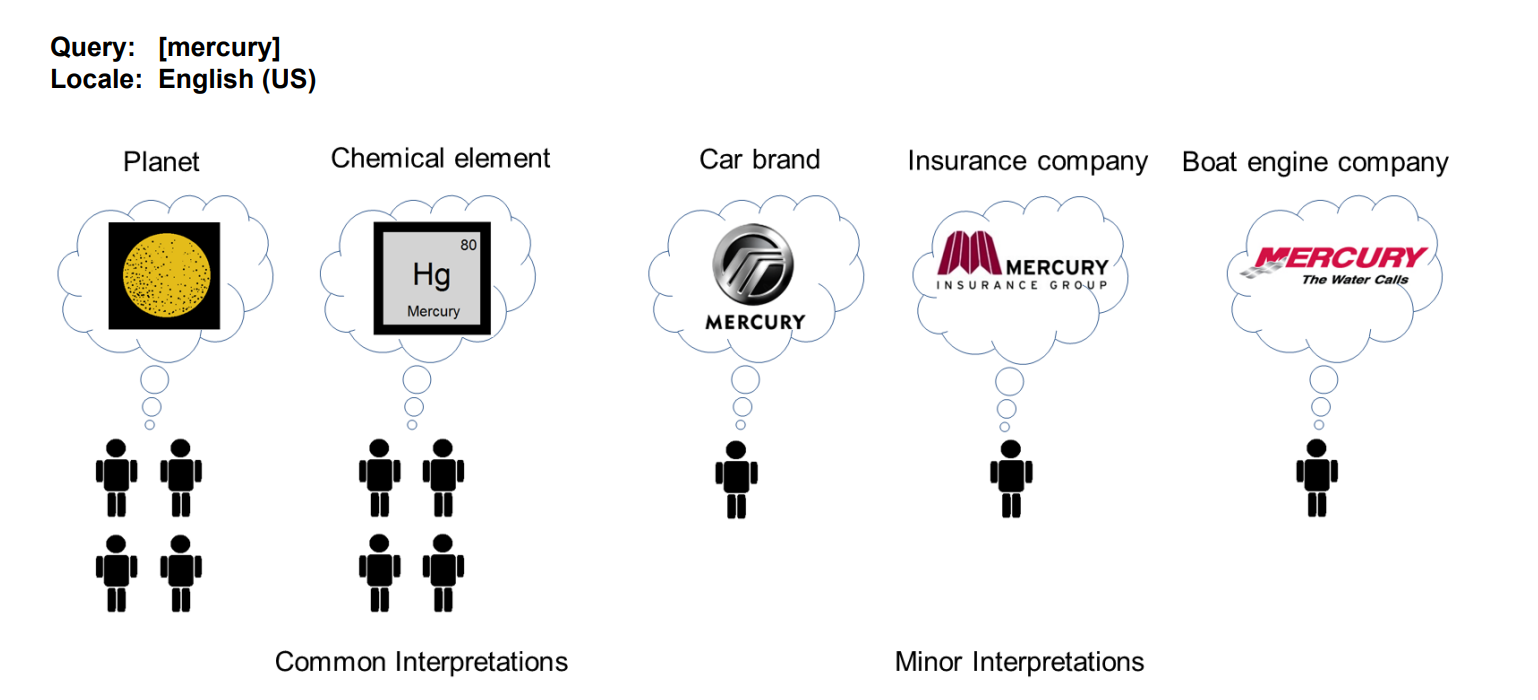
For queries with mixed intent (such as mercury), Google distinguishes between 3 types of interpretation:
- Dominant interpretation: what most people mean when they type in a query. Not all queries have a dominant interpretation.
- Common interpretation: what a significant proportion of users have in mind. A query may have multiple common interpretations.
- Rare interpretation (minor interpretations): what a small number of users have in mind.
When asked “mercury”:
- Dominant interpretation: planet
- Common interpretation: element
- Rare interpretation: car manufacturer, insurance company, boat engine company
We can see the effect of this attribution of intent, for example, with the query ‘mars’, where Google displays different results:
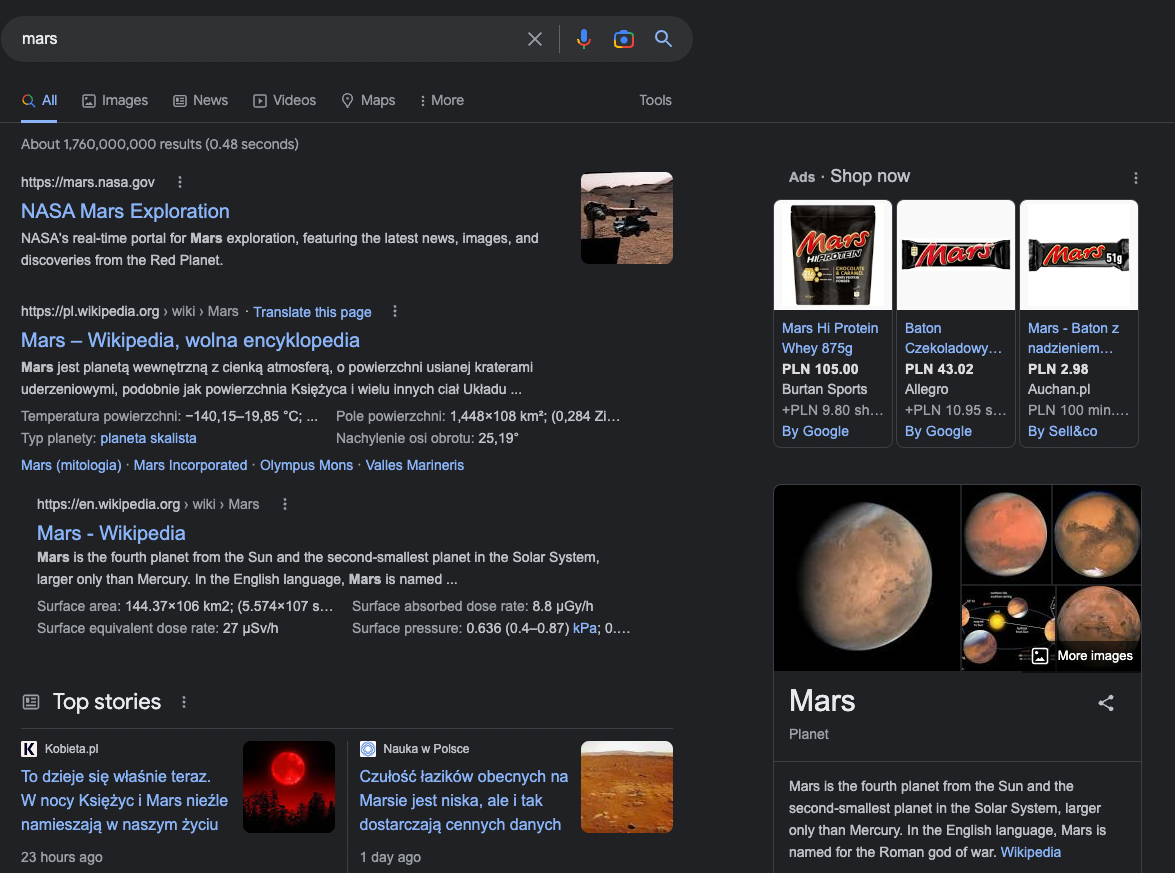
- Dominant interpretation – planet
- Common interpretations – god of war, chocolate bar
It is also worth knowing that intention can change over time.
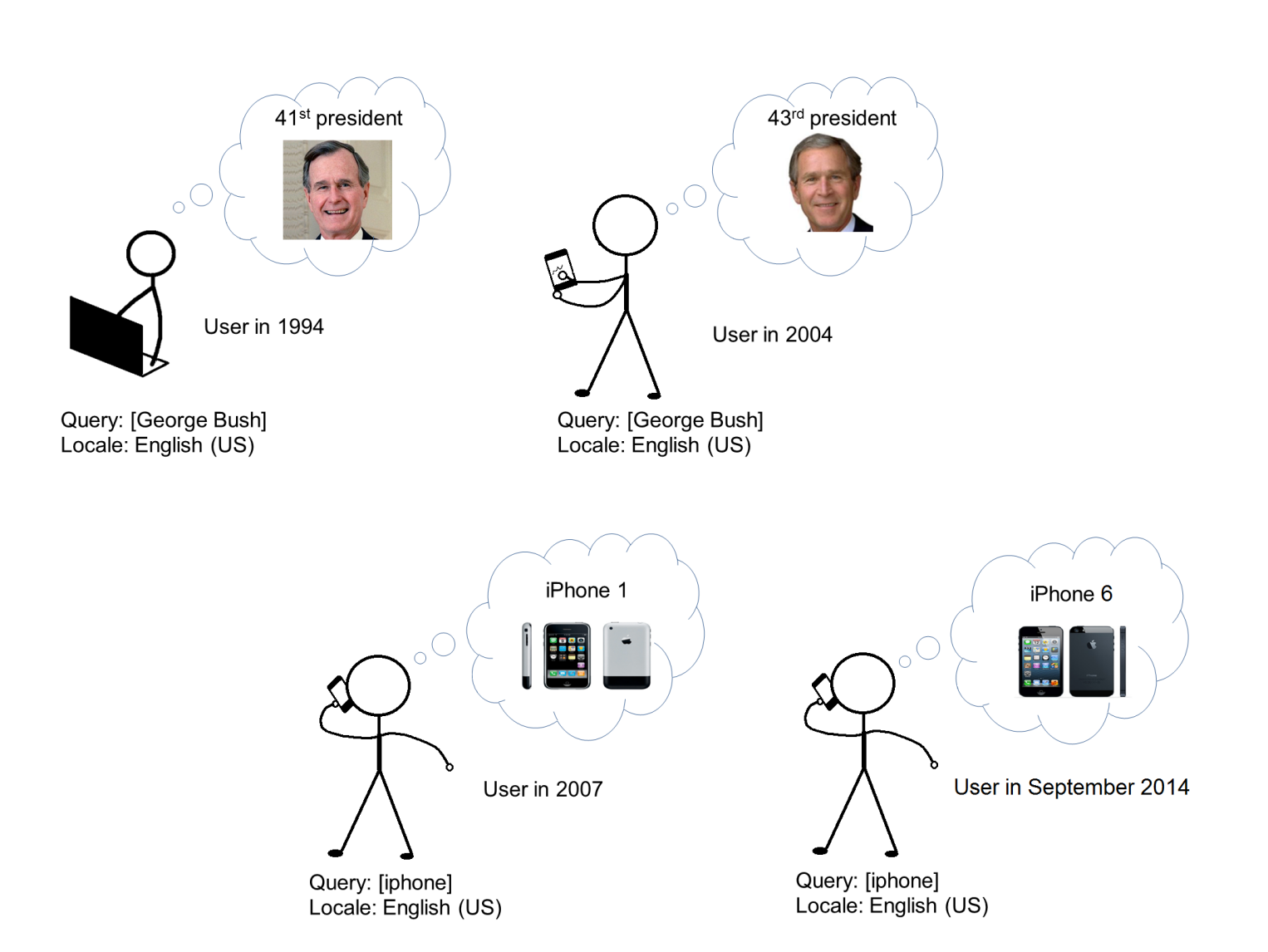
A user typing in the query “iPhone” in 2007 had a different intention (finding an iPhone 1) than in 2014 (finding an iPhone 6). There are many more such examples:
- Independence Day in the USA – at some times of the year the dominant intention may be the title of the film, at others the holiday.
- PlayStation – during Black Friday and Christmas, the dominant intention will be shopping intention (Google will display more shops) and at other times the dominant intention will be research.
The technical aspects of determining intent are described in Google’s patent.
How does Google satisfy user intent?
Google does not only satisfy the user’s intentions by displaying best-fit results. Today, augmented results (snippets) also play a major role. The most common snippets include:
- Direct answer
- Local results
- Opinions
- Sitelinks
- Video
- Top Stories
- Images
- Knowledge panel
- FAQs
- People Also Ask
- Related enquiries
- Google Flights
- Hotel Pack
- Job opportunities
- Google Ads
- Product Listing Ads
Extended results are becoming more common every year. In many cases they are a nightmare for SEO specialists – but for users they are very helpful. The times when there were 10 blue links in the search results (“pure” links to pages, not snippets) are irrevocably gone. I have now counted as many as 1 598 unique ways of displaying search results in Polish search results.
An example of how Google uses my location and snippets to satisfy my intention to check the current weather:
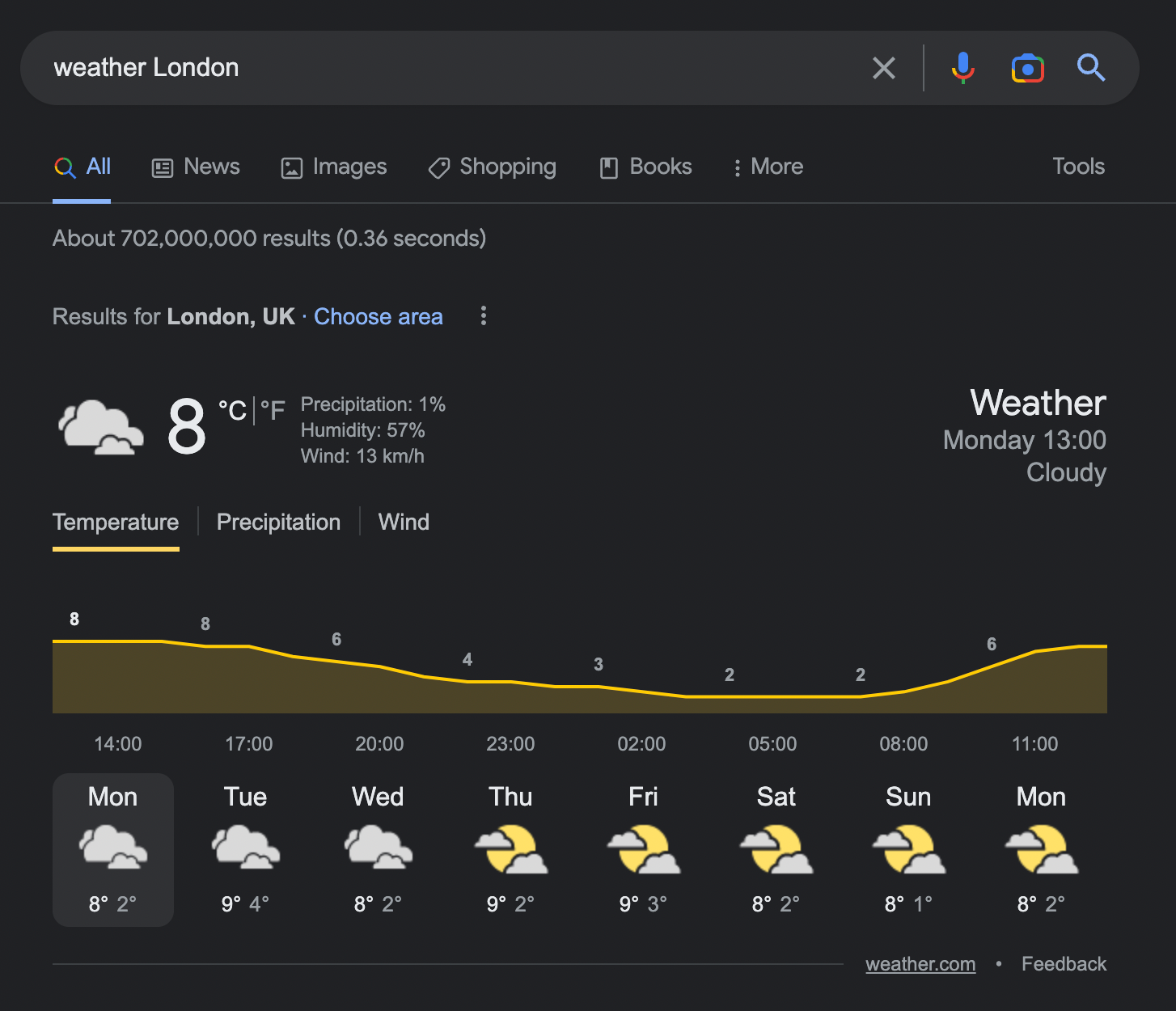
Why does Google rely on snippets?
According to Statista, today by far, more than 50% of users are using mobile devices (in some search categories, the ratio exceeds 90%). Users on mobile devices need fast access to information.
Hence, the snippets and various augmented results that Google now serves us have evolved with the development of mobile devices.
The development of voice search is also influencing the development of enhanced results. With voice communication, the user expects a direct answer – and this is what he or she gets in an increasing number of queries. As a result, Google is said to be ceasing to be a “search engine” and starting to be an “answer engine”.
Methods for identifying intentions
An important task in SEO today is to match the information on a website with the user’s intent. The first step to take this is to identify it. There are many methods to do this, I will briefly discuss the ones that I think are the most interesting.
- Use of snippets – Google, by the way it constructs the appearance of a given search result, in a way suggests to us what the intention of the query is. If it displays a map result, the intention is most likely to be ‘visit-in-person’, if it displays a snippet of the most common questions, the intention is most likely to be ‘know’.
Intentions by snippet can be classified as follows:
Of course, this way of classification does not guarantee 100% effectiveness. Also bear in mind that queries may have fragmented intent. A template for such cases was shared by Kevin Indig – based on Senuto’s data export, you can specify the intent of your keywords in Google Sheets (in the export from each module, there are snippets next to the keywords that appear in the search results; in the case of the Content Planner module, a ready-made intent is provided).
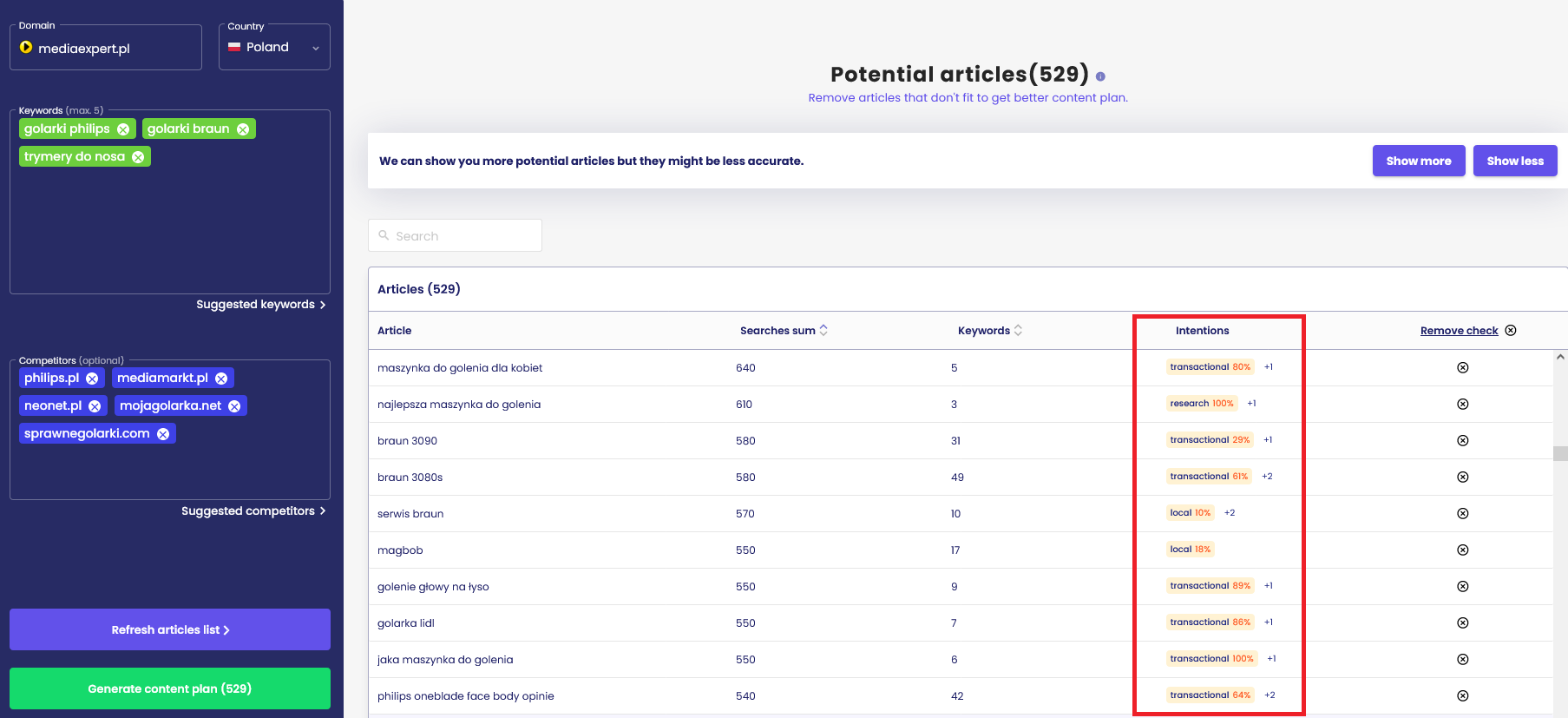
- Dictionary method – words occurring in queries suggest what intention we are dealing with.
For this method, you can use data from Google Search Console and a simple Google Sheets sheet to assign intent. The more accurate dictionary you create, the more accurate the results will be.
3 Artificial intelligence – there are also more sophisticated methods for identifying intentions. For example, the BERT language model that we discussed earlier can be used for this purpose. This is a model that can also be trained to classify intentions. We will not discuss here how to perform this process technically, I leave the website addresses where this is explained in detail.
https://www.kdnuggets.com/2020/02/intent-recognition-bert-keras-tensorflow.html – using the BERT model
https://www.algolia.com/blog/ai/how-to-identify-user-search-intent-using-ai-and-machine-learning/ – using the Glove and Fasttext model (Meta model)
What does an emphasis on user intent mean for SEO?
The fact that Google places so much emphasis on fulfilling user intent has many implications for SEO.
- No match to intent = no position in TOP10 – meeting user intent (however fragmented) is the first step to appearing high in search results. No amount of links or content will make a page not matching intent appear high in search results. Even if it did, it is unlikely to maintain that position for long. If a user has a Know intention (e.g. typing in “how to dress for prom”), the landing page here may not be the page that fulfils the Do intention (e.g. a category page with prom dresses), but rather an appropriately written article with inspiration.
- If the intention is correct, this opens up opportunities to rank for thousands of phrases for a single URL – it used to be that a separate landing page was created for each keyword. Today, thanks to the way the search engine works, a single sub-page can rank high in search results for thousands of phrases if it meets the user’s intention. Google knows that the queries “what to do in Warsaw” and “what to visit in Warsaw” have the same intention.
- Intent is not just about the type of sub-page– many SEO specialists view intent by the type of page that should display in search results (e.g. article, category page, product). You need to think deeper here: 1. Is the user expecting a review, a definition, or perhaps long content? 2. Does the user expect media, videos?
Unfortunately, these questions cannot be answered automatically. Copywriters need to be educated in this area. - Results for fragmented intent – for this type of intent, you need to analyse the search results and decide what type of intent you want to meet: dominant or common? Example: a user types in ‘tools for creating a landing page’ – they may be expecting a product page, a comparison, a list. Google reserves a certain number of places in the results for particular answers, this should be taken into account.
Results for fragmented intent may fluctuate more frequently – results for which intention is not obvious (especially short queries) are subject to fluctuation more often than they used to. It is important to observe such results and react in real time.
Entities
A search engine’s knowledge graph is not built on keywords – it is built on entities and the context of their use. Entities translated into Polish means entities, and the word entities is also often used. As neither of these translations explains exactly what we are dealing with, I will use the English form.
According to Google’s definition, an entity is:
“A thing or concept that is unique, well-defined and distinguishable.”
An example of an entity could be, for example, ‘Robert Lewandowski’. Using the knowledge graph API, we can see how Google stores information about this entity.
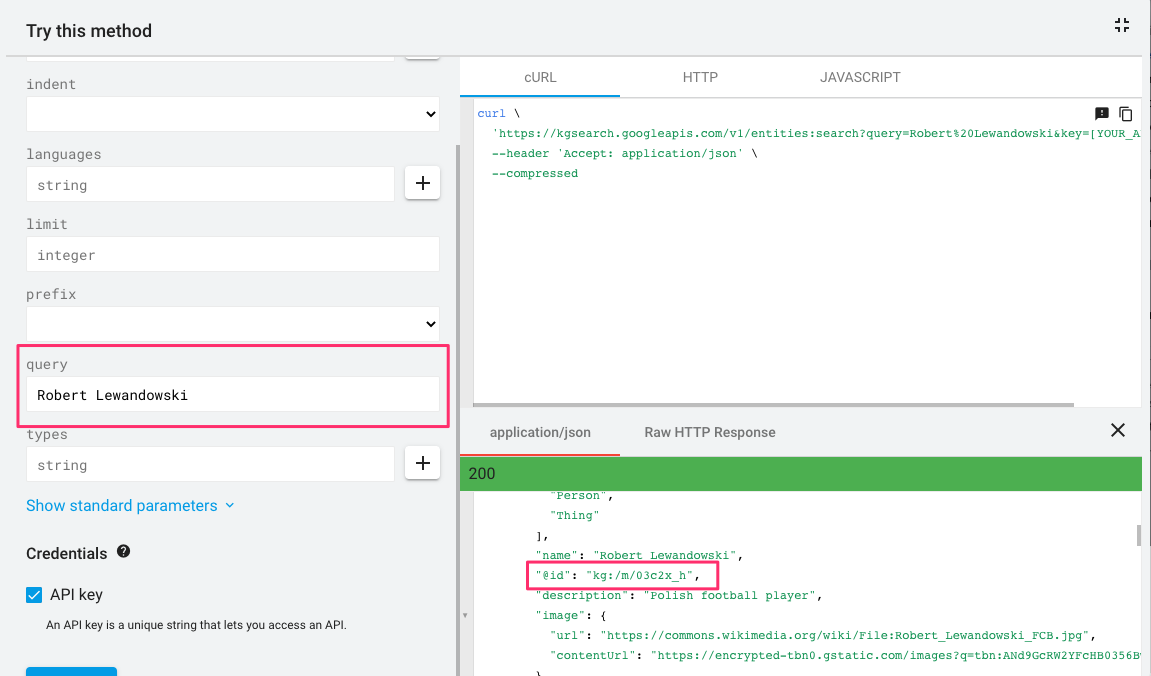
Google knows that Robert Lewandowski is a person, a Polish footballer.
It is worth noting here the @id highlighted in the screenshot. Each entity in Google’s knowledge base has its own unique id, as one query can have multiple meanings (you can check this by typing “castle” as a query, for example).
Entity “Robert Lewandowski” has its own unique id: /m/03c2x_h (things, not strings). An entity with /m/ means that Google added this term to the knowledge graph before 2015, an entity with /g/ means that this took place after 2015.
How does this relate to the user’s intentions (and does an article about Mariusz Lewandowski stand a chance of TOP3)?
When we type the query ‘Lewandowski’ into the Knowledge Graph API we see that there are many results. We have Robert Lewandowski, Mariusz or Janusz. When we type the query “Lewandowski” into the search engine, we are therefore dealing with a fragmented intention. The dominant intention will be revealed in how the search results look, but Knowledge Graph scoring can also be used here.
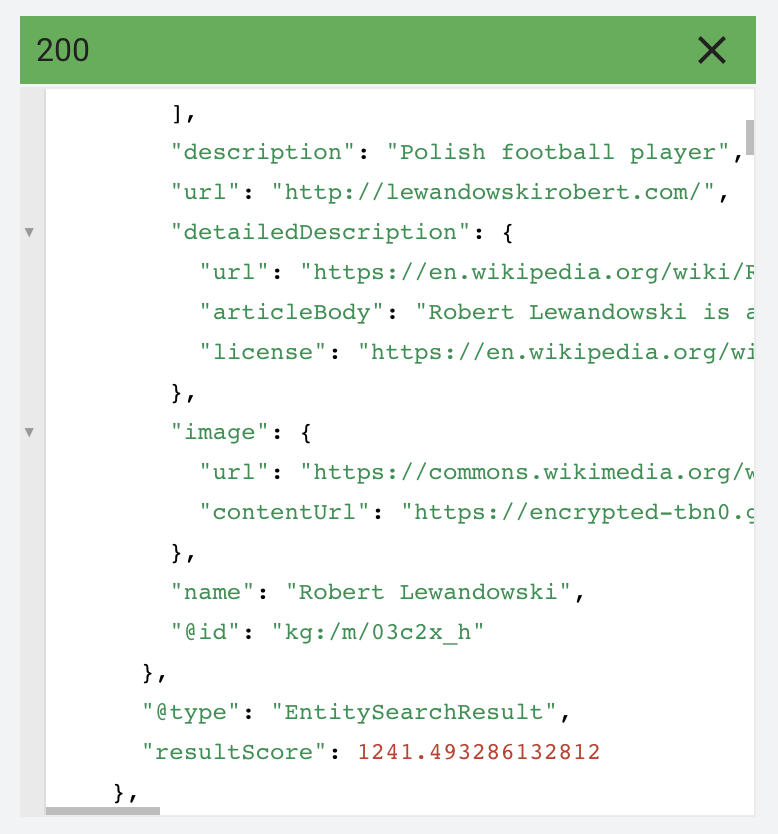
The score for Robert Lewandowski is 1241, for Mariusz Lewandowski (second result) 74. In the TOP50 for this query, it is futile to look for other results. Which means that an attempt to gain a high position with an article about Mariusz Lewandowski would rather fail.
The article is about semantics, and in semantics, relationships are key. The knowledge graph contains relationships precisely between entities.
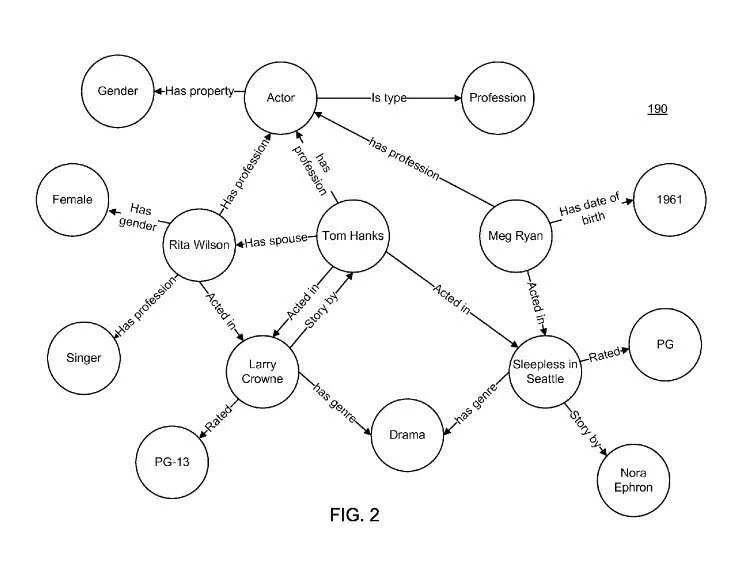
The knowledge graph may contain the entity ‘Tom Hanks’, but also entities describing the films he has starred in and other attributes and relationships (such as gender, age, occupation).
Such a set of features, attributes and relationships for entities is called an ontology. By having millions of unique combinations of features and attributes, a search engine can understand web content and user queries much better.
Google in 2018, in one of its scientific papers, described a possible way to build an ontology base that works for the search engine.
The Biperpedia described in the document is, by definition, a binary attribute ontology containing up to two orders of magnitude more attributes than Freebase (an entity database once used by Google among others, I highly recommend reading this document!).
The screenshot below shows an example of how information is recorded in such a system:
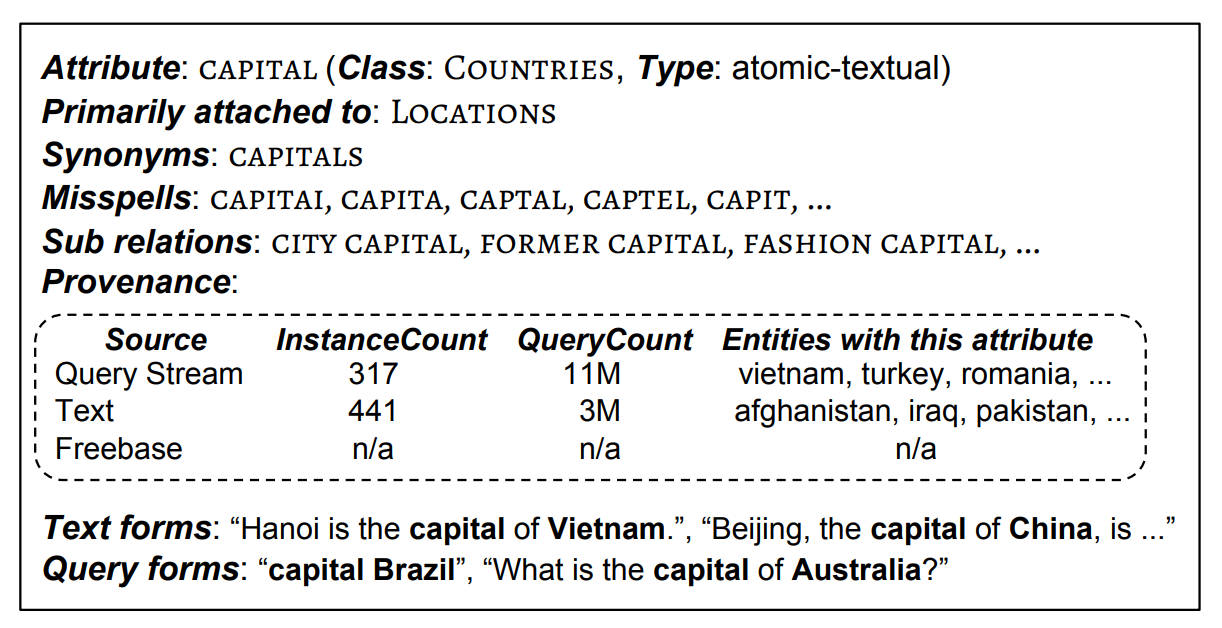
The attribute (in this system it is a relationship of two entities, e.g. capitals and countries) capital is in the set of country classes that are associated with locations.
Synonymous with capital cities.
Incorrect forms of writing are also recorded in the information base.
Additional links: country capitals, fashion capitals.
We also have information about the sources of the attributes:
- User queries – 317 entities contain this attribute, it occurs in 11 million user queries (the system was based on the analysis of 36 billion queries)
- Content from web pages – 441 entities with attribution, included in millions of documents
Below, in the table, you will also find examples of forms extracted from the content and queries.
And here I include some more interesting conclusions from the previously quoted document:
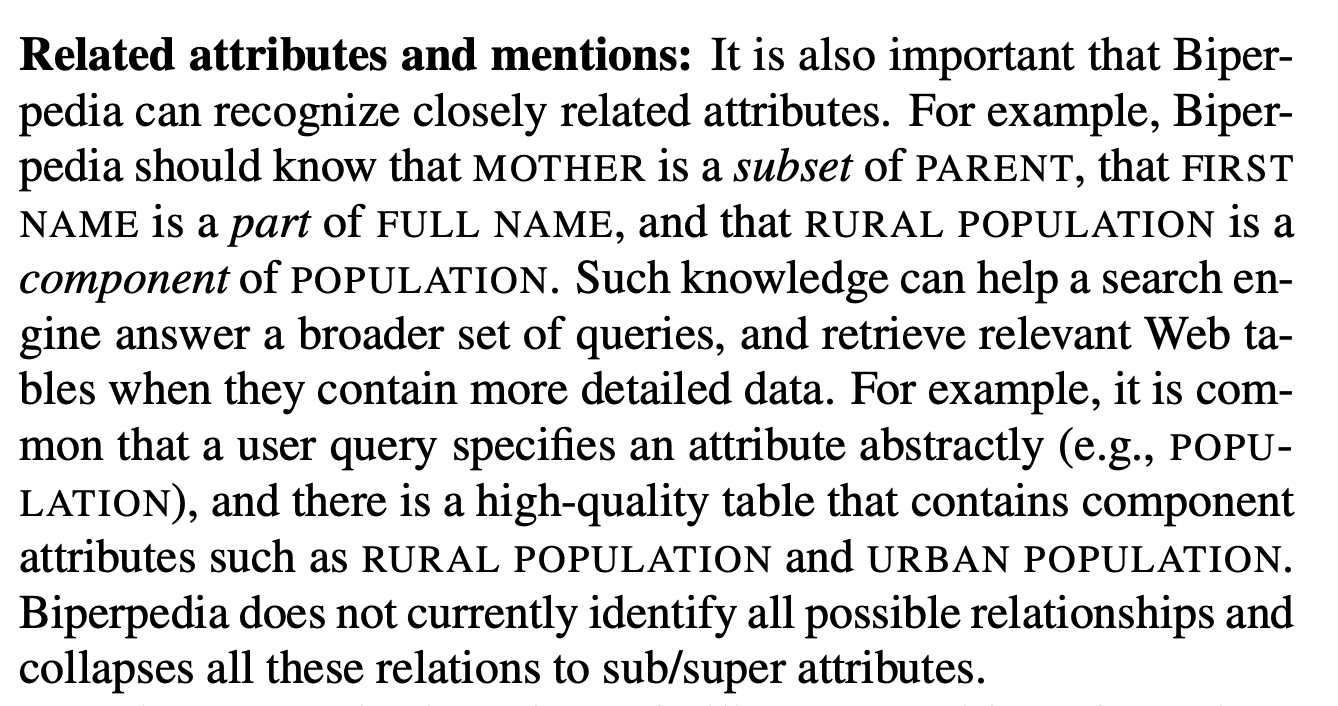
Biperpedia understands the links between attributes (e.g. it understands that the mother belongs to a subset of the parent). This way of storing information allows it to understand broader user queries and return a better result. The whole system focuses on returning formatted information in the form of a ready-made answer – that is why we are talking about a table.
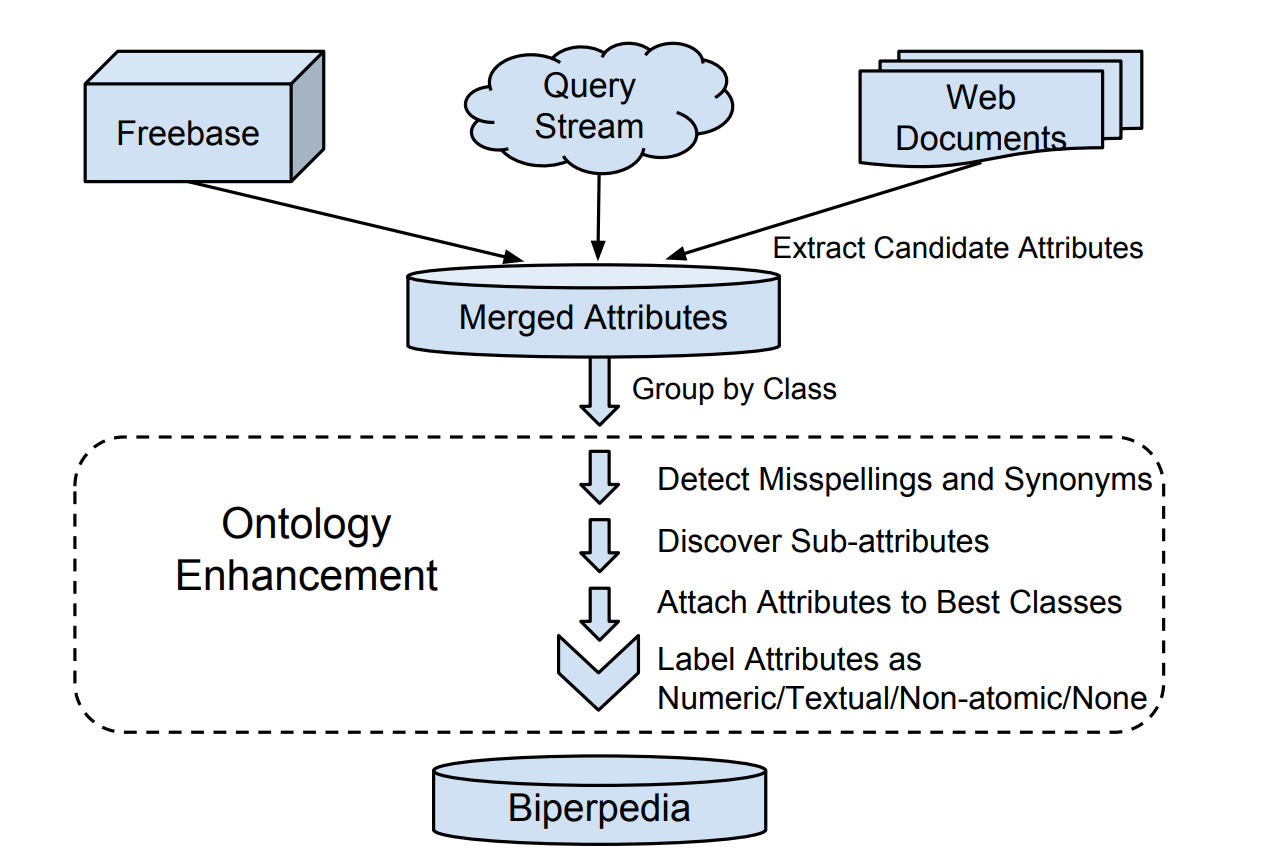
This is how the construction of this base looks like.
- Data is taken from Freebase, Query Streams, websites
- Attributes from these sources are combined with each other
- Synonyms and linguistic errors are detected
- Sub-attributes are detected
- Attributes are assigned to the best classes (one attribute can have multiple classes)
- Attributes are designated by type
- They hit base
Also, section 5 of the document is important (page 4), where there is a description of how the system extracts entities from the content. This extraction is done using the Distant supervision technique. It uses a previously created knowledge base to search for similar elements. In the case of Biperpedia, the most common attributes that already exist in it were used.
The system in its database has the attribute COFFEE PRODUCTION assigned to the entity BRAZIL, when it finds the text ‘Coffee production in Brazil increased by 5%’ using lexical patterns it is able to determine the attribute + entity pair. After content analysis, a set of candidates for the attribute + entity pair is produced.
The system only considers pairs that have a minimum of 10 occurrences (10 occurrences counted in the whole corpus, i.e. in the whole set of documents analysed).
Additional NLP techniques are still used for extraction: parts-of-speech (POS) tagging, dependency parsing, noun phrase segmenting, named entity recognising, Coreference resolution, and entity resolving (if you want to see exactly what each process does take a look at the linked document).
In the final step before the attribute hits the database, a classifier based on a linear regression model does the work.
How have entities made the search engine better?
- Understanding the attributes of entities allows us to display increasingly structured information (snippets, we have more and more results with direct answers).
- The large number of entities attributes allows facts found in the content to be caught, resulting in a better analysis of the credibility of the sources.
- Allows better understanding of very long queries.
Google, by having links between entities, is also better able to meet the user’s intentions. Thus, in addition to the usual results, it is able to return additional information of interest to the user – for example, all the films in which Tom Hanks has starred.
In contrast, when the query ‘Robert Lewandowski’ is queried, Google shows other footballers who are in some way related to Robert.
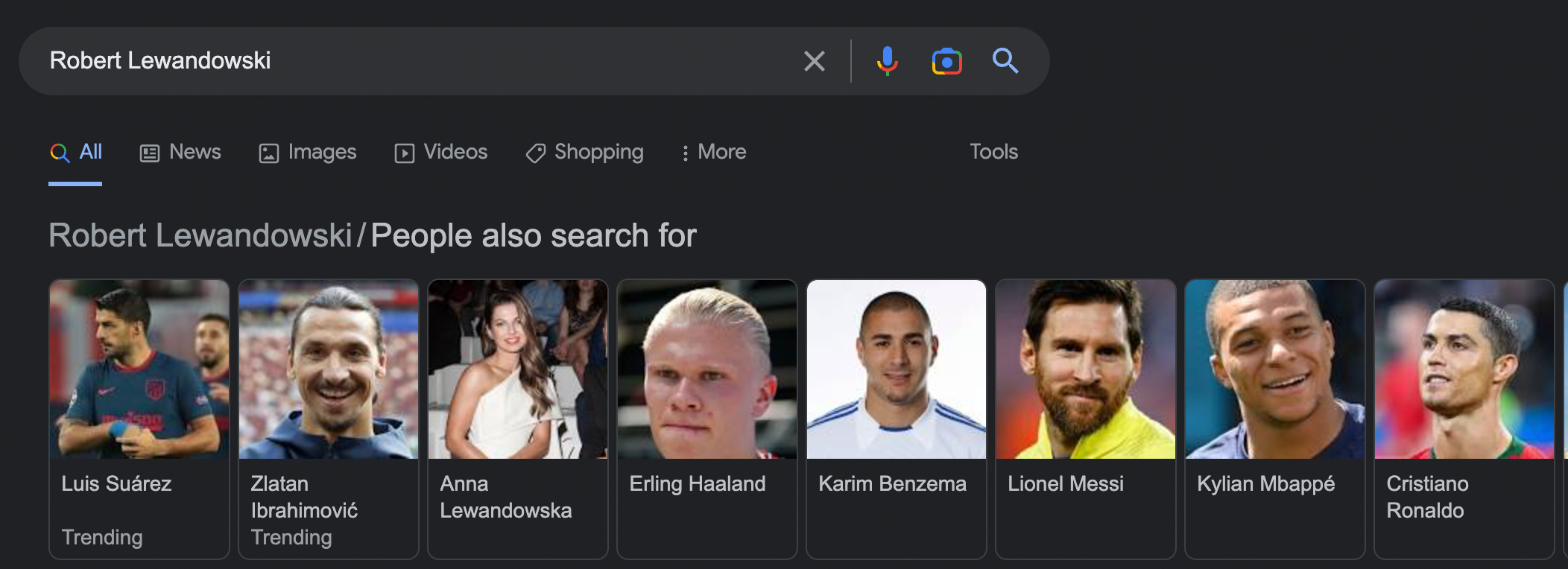
How accurately Google recognises entities can be tested using the Google NLP API. This is an API that allows you to implement NLP algorithms similar to those used by the search engine in external systems. The API itself does not work in Polish (list of available languages), so I will show an example in English.
I used the first four paragraphs of the page about Robert Lewandowski on Wikipedia as text:
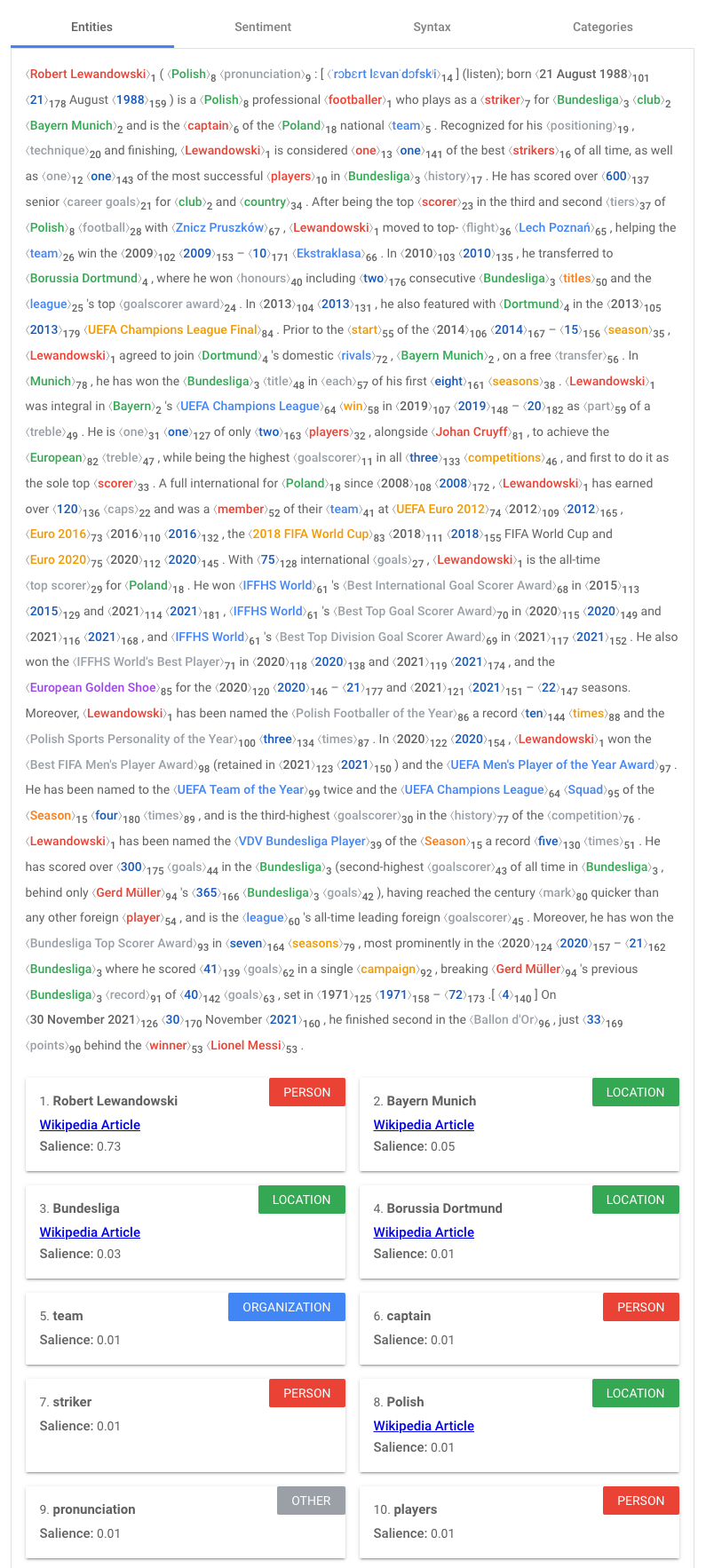
źródło: https://cloud.google.com/natural-language#section-2
Each text highlighted in colour are recognised entities, below is a description of each. From it, we can learn that:
- Robert Lewandowski is a person
- The salience for this entity is 0.73 (a 0-1 scale that determines how relevant a particular entity is in a given document/text)
As can also be seen, in some cases the API is not precise, e.g. Bayern Munich has been labelled as a location, while it is a football club. However, search results indicate that Google understands this entity, which may suggest that the latest models are not used for the API.
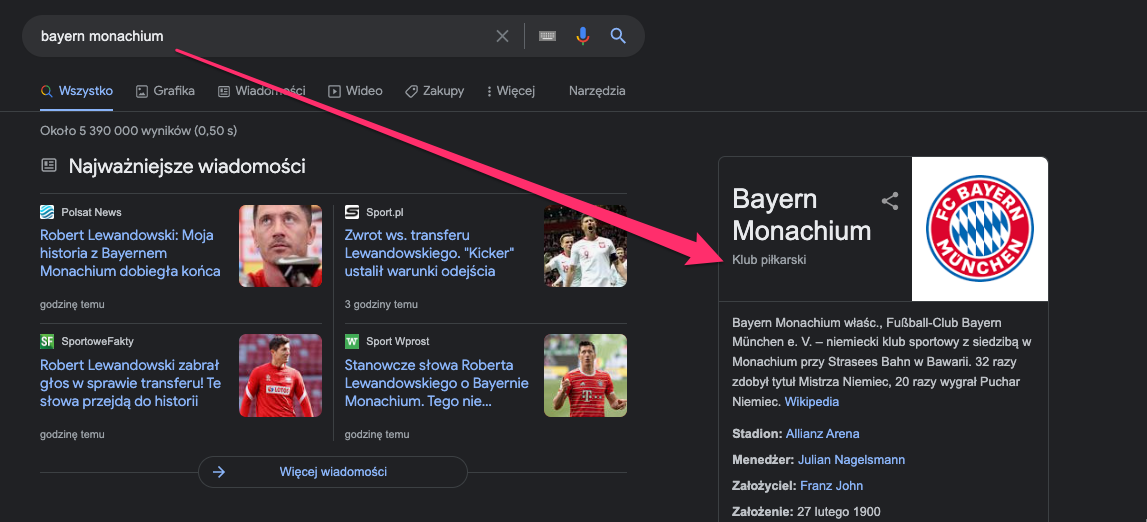
In addition to analysing NLP API entities, Google can do much more – including categorising content. The previously quoted Wikipedia text about Robert Lewandowski fell into two categories:

The full list of categories is available here. And you can check out the API demo here.
The API for up to 5,000 queries per month is free. After that, depending on the features used, you pay between $0.125 and $2 per query.
If you want to use NLP in your project in Polish, then you can use other language models available on the market.
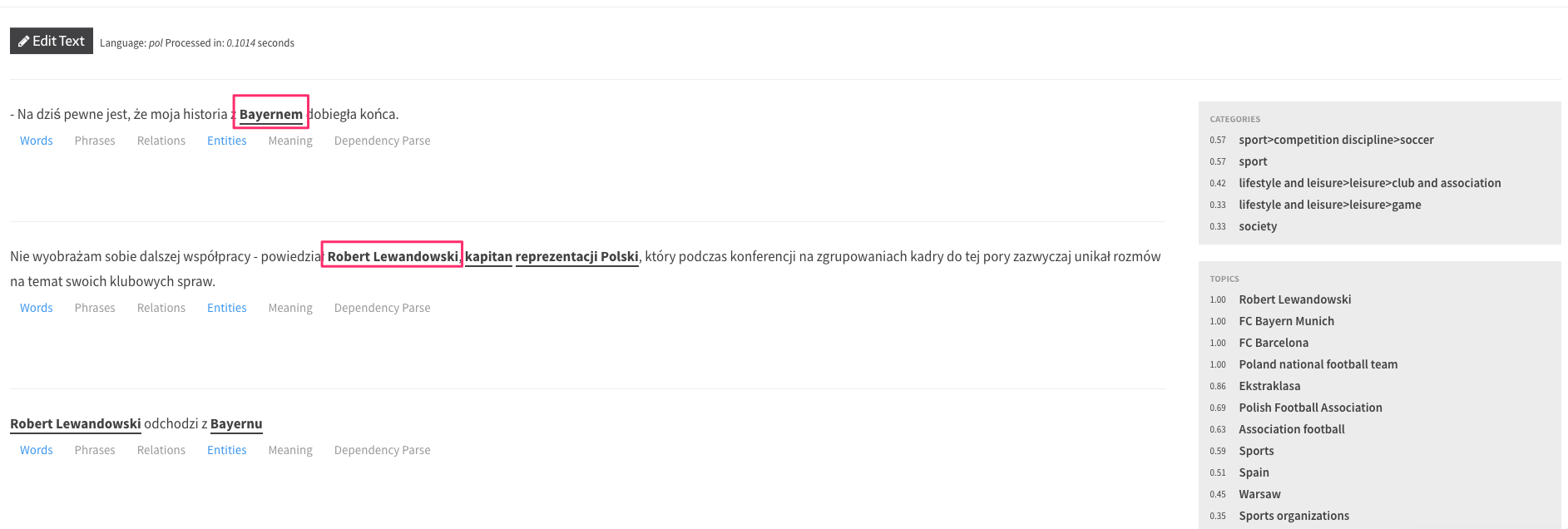
Analysis of entites in Polish by the Text Razor model.
Analysis of entities in Polish by the Text Razor model.
Here are two popular solutions:
The models and libraries themselves are plentiful on the internet.
Entities are important for content creation and information architecture in SEO.
The rest of this article will show you how to put this knowledge into practice.
In the last two years, the concept of topical authority has been making its way very strongly into the SEO world.
Thanks to the aforementioned algorithms, models and changes, Google has stopped looking at sites through the prism of keywords and has started to look through the prism of concepts.
By knowing the relationships between terms, Google is able to determine which terms belong to which topic. This allows it to determine how a site fits into that topic (rather than a specific keyword) – and to assess how expert it is in that topic.
Google, mindful of its mission to fulfil the user’s intention, prefers to refer the user to expert sites where the user will fully realise his or her intention.
We refer to such sites as having high topical authority.
Example
A user types the query ‘SEO’ into a search engine – in the past, Google would match the best documents based on a single query and a number of other factors such as links.
Today, Google understands that the term SEO also includes terms such as links, technical optimisation, sitemap and thousands of others. Sites that fully cover these areas of expertise – answering all these terms as intended – are more likely to appear at the top of the search results.
Of course, this is not the only algorithmic factor and sites must meet a number of other requirements, but topical authority is very important today. E.g. by building expertise it is possible to be high in the search results without links – but it is hard to be high in the search results just by building links, excluding the element of expertise.
However, we need to keep the intention in mind at all times here. Sometimes the user’s intention does not require the site to be expert on a particular topic, because the intention itself is superficial. In such cases, topical authority will not play a key role.
John Muller of Google commented on topical authority.
Here are two quotes from John’s statement:
“When we want to rank for a specific topic on Google is it a good practice to also cover related topics. For example, if we sell laptops when you want to rank for that is it useful to create posts like reviewing laptops, the introducing the best new laptops, those kind of things? And if it’s useful then it doesn’t have to be done in any special way?”
“So I think this is always useful because what you’re essentially doing is on the one hand for search engines you’re kind of building out your reputation of knowledge on that specific topic area. And for users as well it provides a little bit more context on kind of like why they should trust you. If they see that you have all of this knowledge on this general topic area and you show that and you kind of present that regularly, then it makes it a lot easier for them to trust you on something very specific that you’re also providing on your website. So that’s something where I think that that always kind of makes sense.
And for search engines as well. It’s something where if we can recognize that this website is really good for this broader topic area then if someone is searching for that broader topic area we we can try to show that website as well. We don’t have to purely focus on individual pages but we’ll say oh like it looks like you’re looking for a new laptop, like this website has a lot of information on various facets around laptops.”
To summarise:
- John says that if you want to rank high in Google for a topic, it’s also worth writing content on related topics.
- Doing it this way builds a ‘reputation for knowledge in a particular area’ – he mentions topical authority here.
- This is also important for users who, with more context, gain more trust.
- With this approach, Google can narrow down its search area – instead of analysing the best pages for each query, it can provide the user with a set of pages that best fill a concept.
For my presentation at the 2021 SEO Festival, I examined the correlation between topical authority and position in search results.
Survey methodology:
- I considered 212,000 keywords in the study,
- Using Senuto’s clustering method, I divided these keywords into 7,200 semantic groups,
- For each keyword, I checked the TOP10 results and examined how many keywords among those assigned to a semantic group they address in their content (one semantic group should correspond to one subpage). Based on this, I arranged a metric on a scale from 1 to 100: 1 – very poor coverage, 100 – full thematic coverage.
The results are as follows:
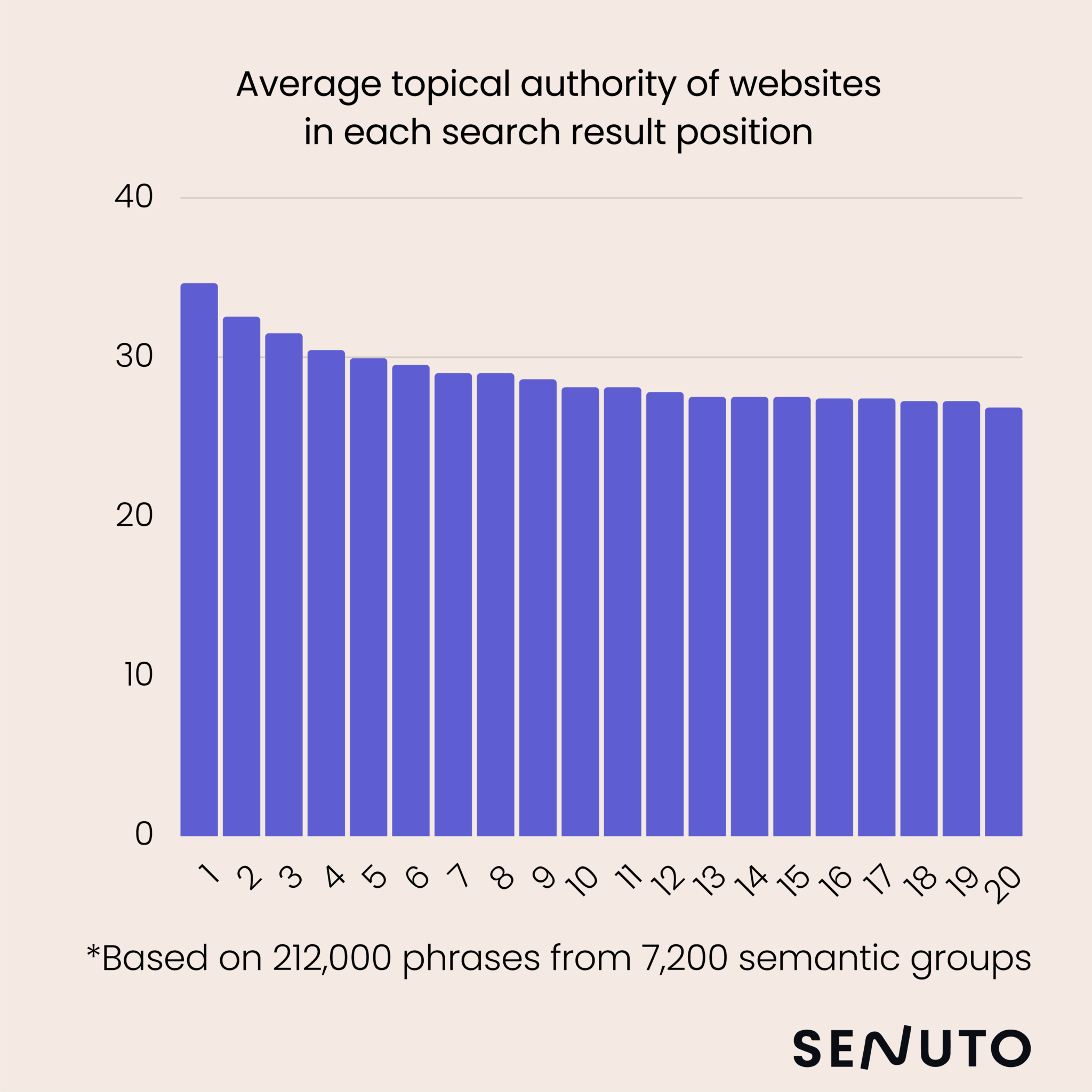
As you can see, the higher the position, the higher the topical authority. I also examined the correlation between position and high topical authority. The correlation result is 0.69, which means that there is a correlation.
Of course, I realise that the research method here is not ideal, as I am examining individual sub-pages instead of entire sites, plus the study is in isolation from other factors such as the number of links or the level of optimisation of the site – but this is the only method I had available to me.
Leaving aside the study itself, we can refer to observations of how the search engine has changed in recent years. All of the significant updates to the algorithm relate to how Google analyses content and intent matching, meaning that factors in this category are important to the search engine, and will strongly benefit from this relevance in the future.
What else is worth knowing in the context of semantic SEO?
For a full understanding of semantic SEO, you need to understand a few more mechanisms of how the search engine works. We will go through them one by one and then you will already have the full picture in your head.
Assessment of the comprehensiveness of the information provided
In line with the concept of topical authority, Google wants to refer the user to comprehensive sources of information. To this end, it evaluates each document for additional information that the user may not have found in other documents (e.g. a blog post that comprehensively explains the nuances of the keto diet and is richer in knowledge than posts on competing blogs).
Google’s patent states that:
Techniques are described herein for determining an information gain score for one or more documents of interest to the user and present information from the documents based on the information gain score. An information gain score for a given document is indicative of additional information that is included in the document beyond information contained in documents that were previously viewed by the user. In some implementations, the information gain score may be determined for one or more documents by applying data from the documents across a machine learning model to generate an information gain score. Based on the information gain scores of a set of documents, the documents can be provided to the user in a manner that reflects the likely information gain that can be attained by the user if the user were to view the documents.
In practice, this means that Google can increase the positions of sites that provide new information for a given user query and decrease the positions of sites that do not contribute any new information.
Nowadays in SEO, most content is created based on an analysis of the current TOP10 search results. This is not a bad technique, but it often pushes you into the trap of duplicating already existing information.
Our aim should be to create content that contributes more information than the pages currently high in the search results.
In practice, this means, among other things, that in the process of keyword research, we should not select only those phrases that have searches. Those that do not have them should also be developed by us (and therefore used in the content). In this way, there is a chance to close the information gap and rank higher in the search results.
More has been written on this subject by Bill Slawski.
Site information architecture & internal linking versus semantic SEO
From a semantic SEO point of view, there are two ingredients for success:
- Writing content in such a way that it covers the topic and responds to the user’s intentions.
- Organising it on the website in such a way that content that coincides thematically is close together in the structure and the structure itself is understandable to the search engine – and this is the aspect of properly laid out information architecturethat we will now discuss.
Properly structured information architecture affects many aspects of SEO (such as crawl budget), here we will consider it from a semantic SEO point of view. The key is to keep similar content in the site structure close enough together. We will introduce two concepts here:
- Topic clusters – semantically convergent content (e.g. an article on semantic SEO and an article on internal linking).
- Pillar pages – according to this technique, we should have one article that covers a topic in a very comprehensive way, and many articles supporting it with additional information.
A diagram of such an architecture could look like this:
The diagram shows an illustrative part of the structure. A realistic structure that would achieve high topical authority would have to be much more elaborate.
This structure is also often referred to as a silo structure.
- At the top of our diagram, we have a general article on SEO – this is our pillar page.
- Lower down we have the Level 1 cluster, which still addresses general issues, but these are part of the knowledge contained in the pillar page.
- Lower down we have the level 2 cluster, which already addresses very specific issues, also derived from knowledge from cluster 1.
There are also internal links between the various sub-pages. These are not in every case a necessity and should not be added by force. Such an organised structure will allow the search engine to understand the site better and analyse it faster. This in turn will translate into an improvement in many SEO parameters (e.g. crawl budget).
At this point, you may be asking yourself quite a few questions, and I answer them below:
- Can I add internal links between clusters?
Between clusters organised under one pillar pages, as much as possible. Between clusters organised under other pillar pages, not always. If they are far apart thematically, try to separate them (e.g. Health and Construction), but if they coincide thematically, you may add such links (e.g. SEO and Google Ads).
- What type of internal linking is best?
The best from a semantic SEO point of view will be links from content. By surrounding the link with content, the search engine has the necessary context when analysing it.
- How many internal links to add per cluster?
There is no golden rule. Remember that the more internal links you add, the lower the power of a single link. If a site has 300 internal links, each one conveys 1/300 of its power. That being said, it is worth following some good practices:
Avoid multiple repeated links in the footer (so-called site-wide links) – for example, if you run a shop and a blog, the menu from the shop should not appear on the blog
– Add only related clusters in widgets and suggested links.
– Use as many links in your content as make semantic sense. Do not apply rigid quantity rules.
- Should the URL include the entire path to the cluster, e.g. domain.com/seo/technical-seo/404
With the right internal linking, Google can handle the analysis of such a structure, especially if you use breadcrumbs in addition, for example. However, maintaining such a directory structure is always an advantage. Both from a search engine and user point of view.
- Can I create further level clusters?
As a general rule, it is a good idea to keep the site structure fairly flat. This makes it easier for the search engine to crawl, and also allows you to better manage PageRank flow. However, if the topic you are operating in is very deep (e.g. health) you may need to create another level of clusters.
A good example to follow here would be the Webmd service, for example. Let’s look at how they meet the requirements of semantic SEO.
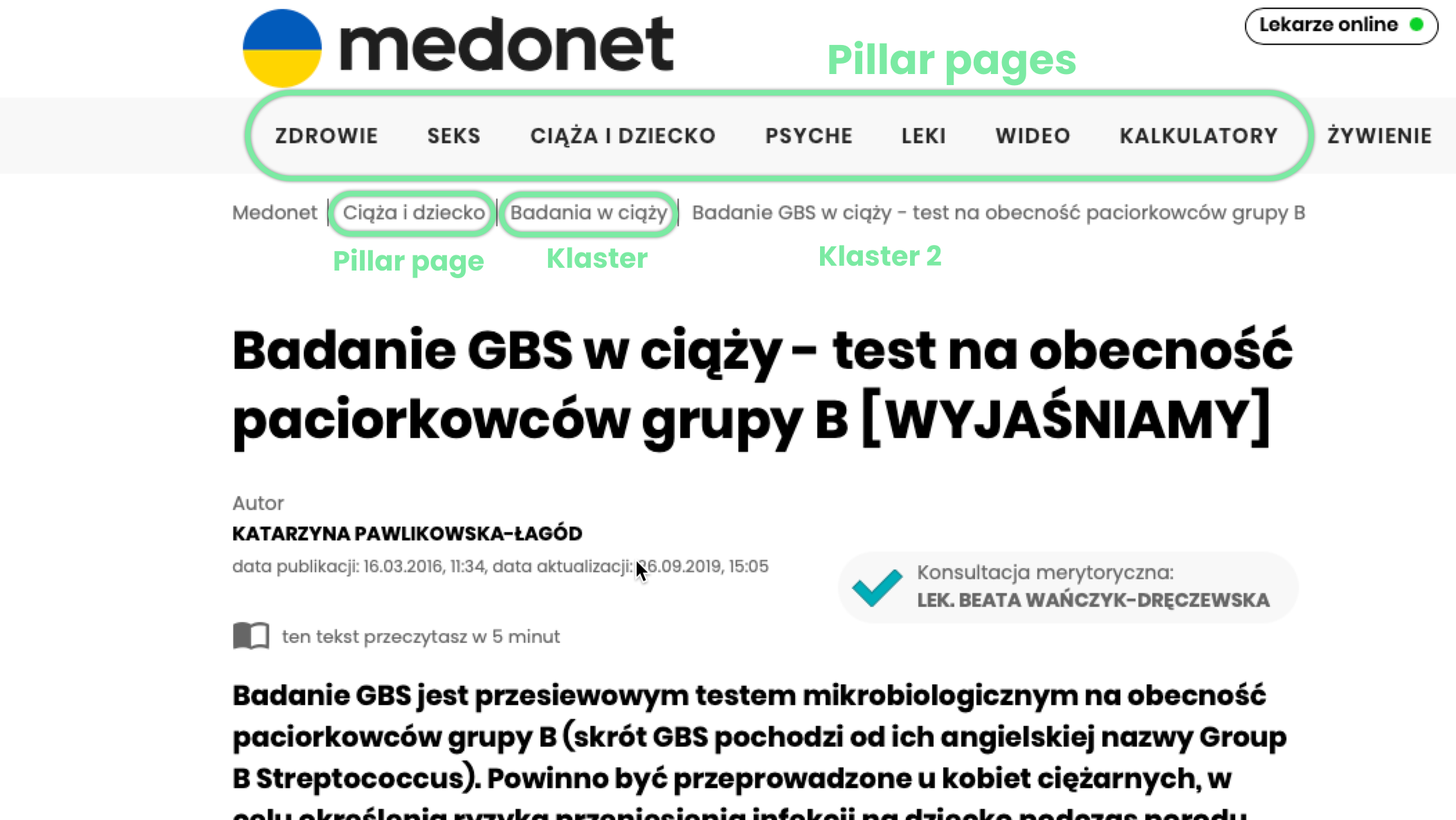
- Medonet organises the architecture around pillar pages (Health, Sex, Pregnancy and Baby).
- Each pillar page has its own content (https://www.medonet.pl/ciaza-i-dziecko,kategoria,157.html) that links to the clusters
- Uses clusters linked to the pillar page that describe the topic in more depth
URL structure and breadcrumbs convey the full path to the lowest level cluster - The information architecture is quite flat, everything is at most a few clicks away from the home page
- All internal links are closely linked to the cluster (as in the screenshot below)
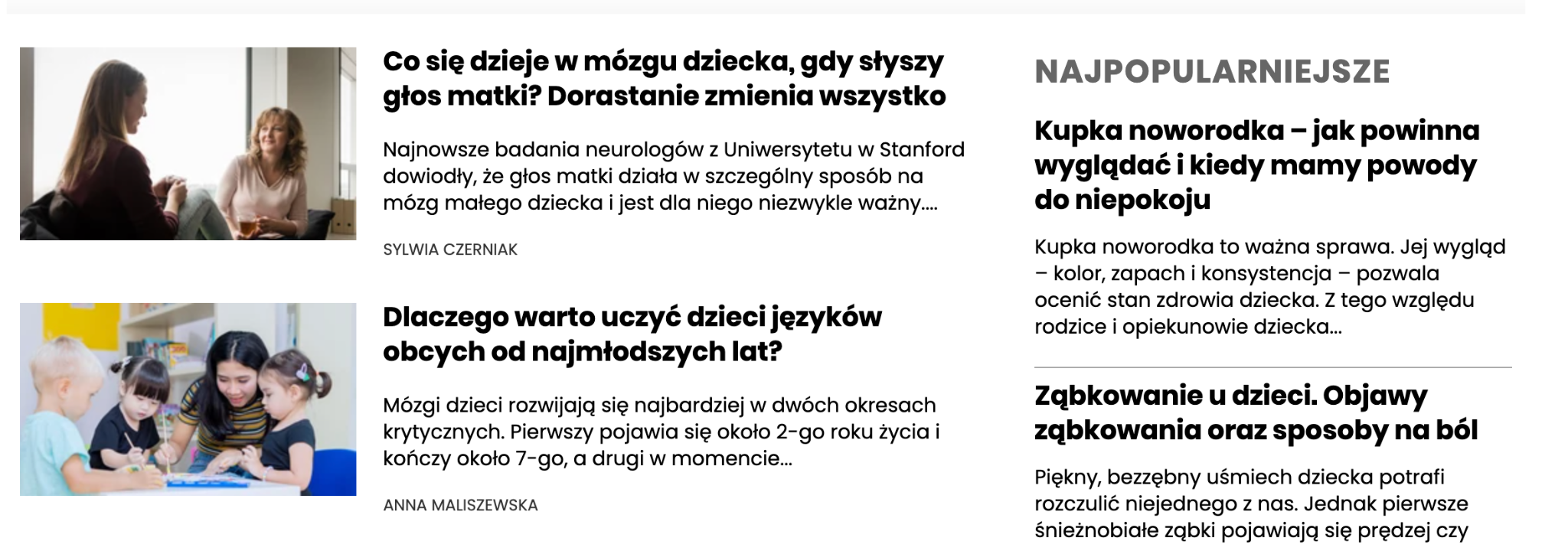
https://www.medonet.pl/ciaza-i-dziecko/rozwoj-dziecka,kategoria,164.html
This has resulted in Medonet’s very high positions in search results, which have been maintained for a long time.
A second, not entirely good example is the website zdrowie.wprost.pl.
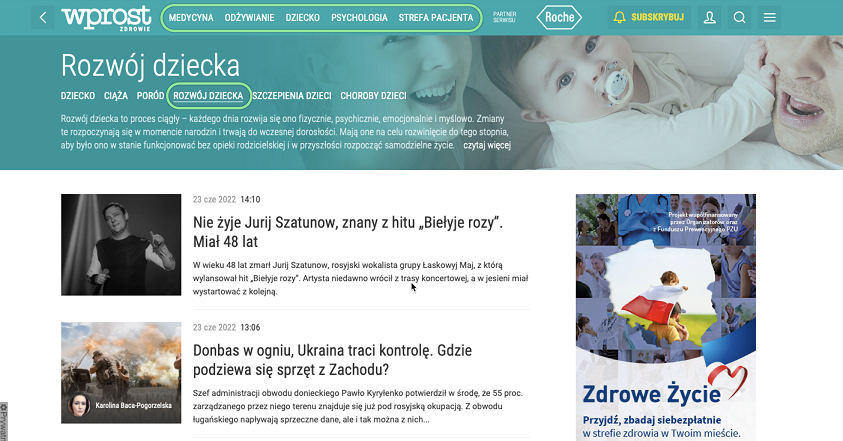
źródło: https://zdrowie.wprost.pl/dziecko/rozwoj-dziecka
Admittedly, at first glance the structure seems similar to Medonet, but:
- The pillar pages are empty (e.g. Child Development only contains links to articles)
- Neither the URL structure nor the breadcrumbs convey the path
- Internal linking is poorly organised. The cluster about child development contains very many unrelated links (screen below)

Despite the fact that the website zdrowie.wprost.co.uk has, in theory, more pages indexed in the search engine, its visibility is almost 10x lower.
I wanted to take this opportunity to invite you to check out our internal linking automation offer. Vestigio uses a proprietary algorithm to provide you with a ready-made recipe for internal linking within your website. For you, this is an essential support on the road to higher rankings in Google. Click the banner below.
How important it is to update content in semantic SEO
There has long been a perception in the SEO world that Google favours sites that frequently add new content and update the content they have already published.
In terms of content updates and semantic SEO, two Google patents are relevant:
First to Document scoring based on document content update.
The patent reads:
A system may determine a measure of how a content of a document changes over time, generate a score for the document based, at least in part, on the measure of how the content of the document changes over time, and rank the document with regard to at least one other document based, at least in part, on the score.
From this it can be concluded that:
- Google can examine changes to a document over time and create a score for the document in question on this basis.
In the patent, we can also read out according to which formula the assessment is calculated.
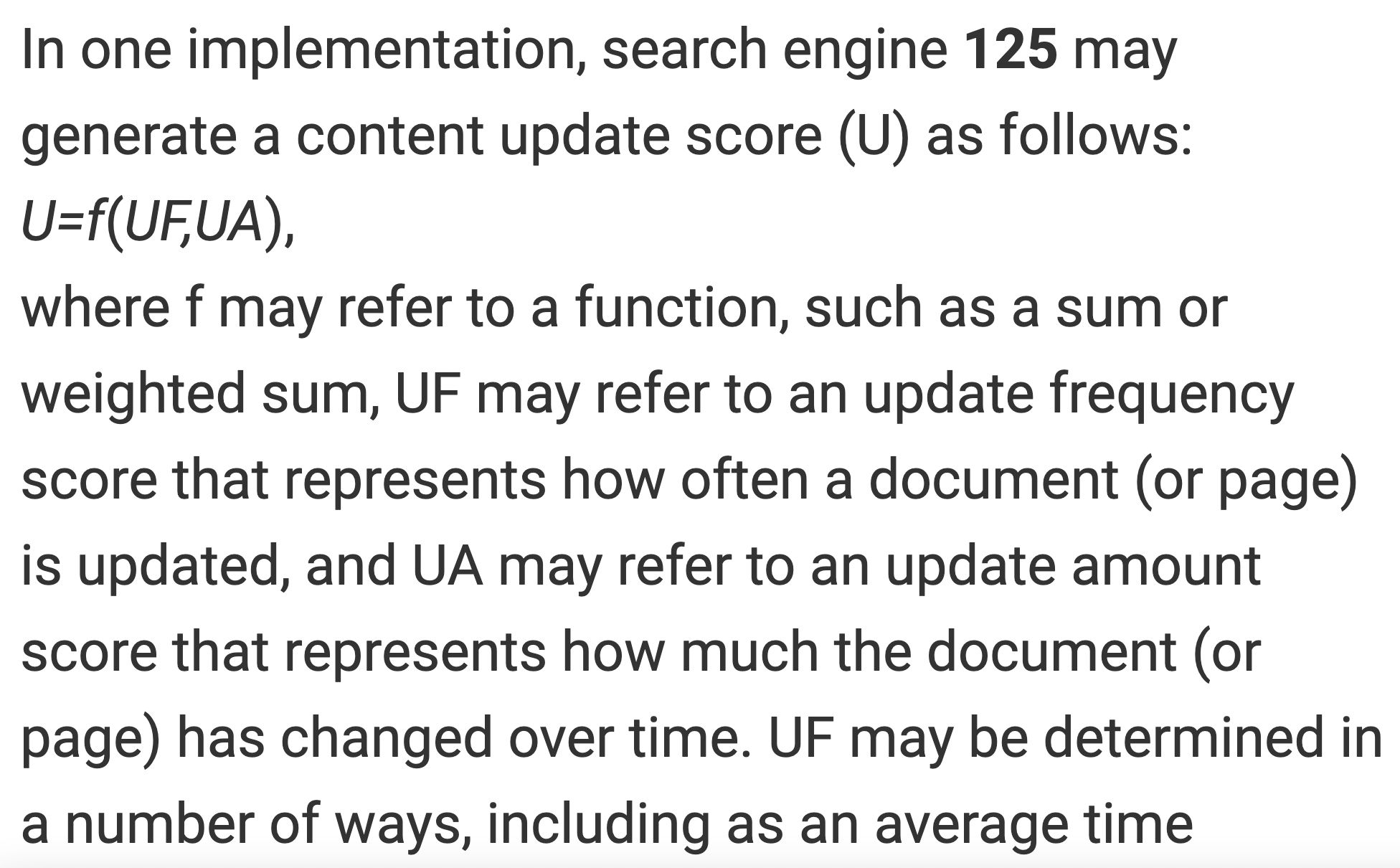
The U (content update score) consists of three elements:
- f – sum, which specifies that the formula may refer either to a single document or to a number of documents in total
- UF – defines how often the document is updated
- UA – indicates how much the document has been altered
What does this mean in practice?
- Sources that are updated more frequently can gain positions in search results.
- The more content we add, the higher the scoring our site will get – adding new content will also positively influence those added earlier (as long as they coincide thematically).
- It is worth updating old content, especially content where the user’s intention may change over time.
In terms of changing intent over time, there is a second Google patent: Query Deserves Freshness (QDF).
By definition:
QDF (Query Deserves Freshness) – a mathematical model that tries to determine when users want new information and when they don’t. (Model matematyczny, który stara się określić, kiedy użytkownik chce, a kiedy nie chce otrzymać nową informację.)
Based on analysis of blogs and magazines, news portals and query analysis, Google assesses whether users for a given query are expecting new content. In the case of such queries, a Google News snippet usually starts to appear in the search engine, while Google may also then update the normal search results.
What are the implications of this?
It is worth keeping an eye on trends and updating that content, which may require new knowledge.
Myths in the area of semantic SEO
Many myths have grown up in the field of topical authority and semantic SEO. I would like to address them in this part of the article.
1. Content length matters
In many articles, even those dealing with topical authority, we will come across the statement that an important factor in building authority is the length of content and that it is one of the algorithmic factors.
After reading the content of this article, you have probably guessed that this is not the case. The length of the content in itself does not matter to the search engine.
The content should be as long as the user requires. In some searches, the user’s intention is to read long content, in others it is not. In some cases, content that is too long may even lower the position.
I conducted a study to prove my thesis.
- In the study I considered 209,411 phrases
- I examined content posted on 66,072 unique domains
This is the average length of content (in characters) in the individual positions in the TOP10 search results:
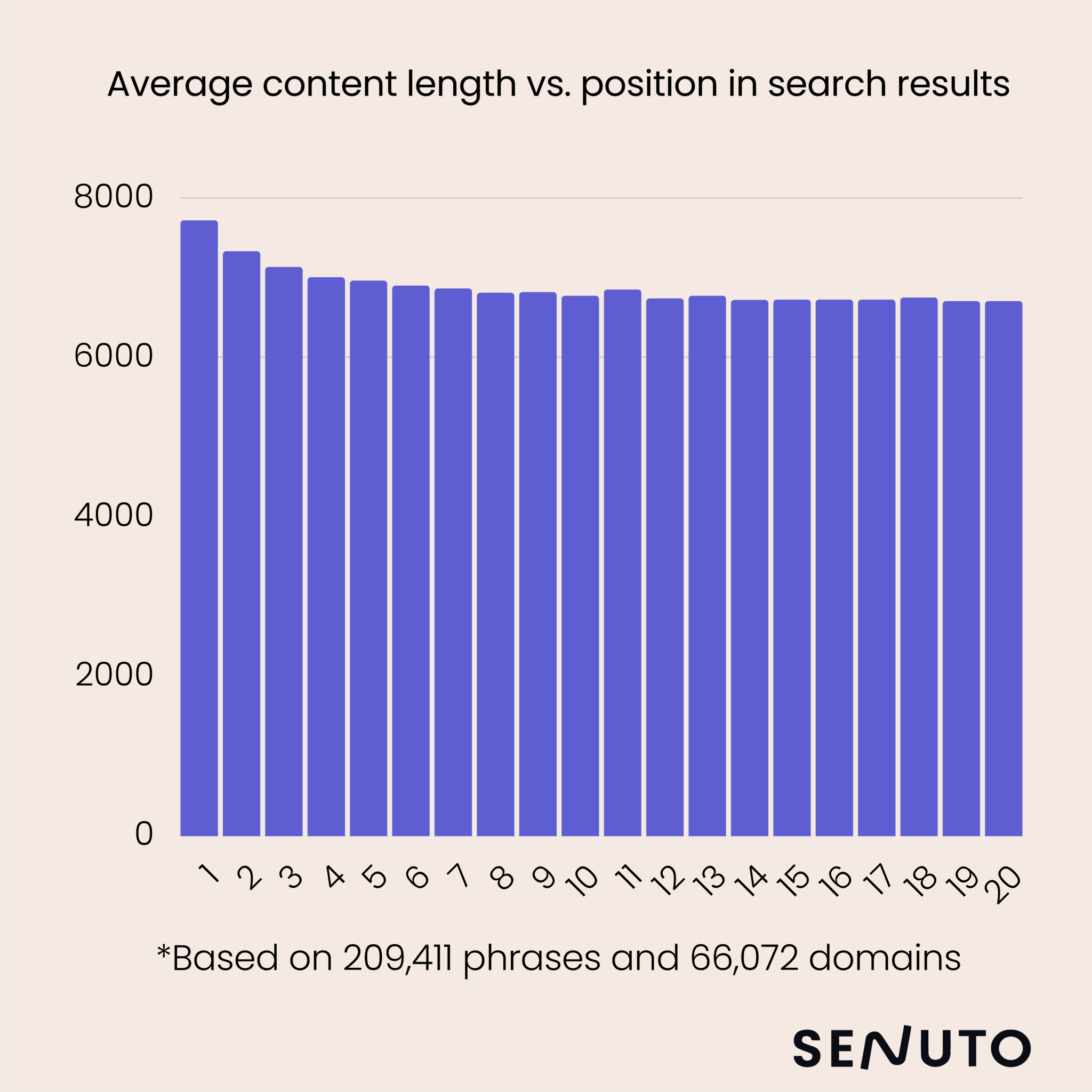
And this is how the % of longest texts in each position in the search results is presented:
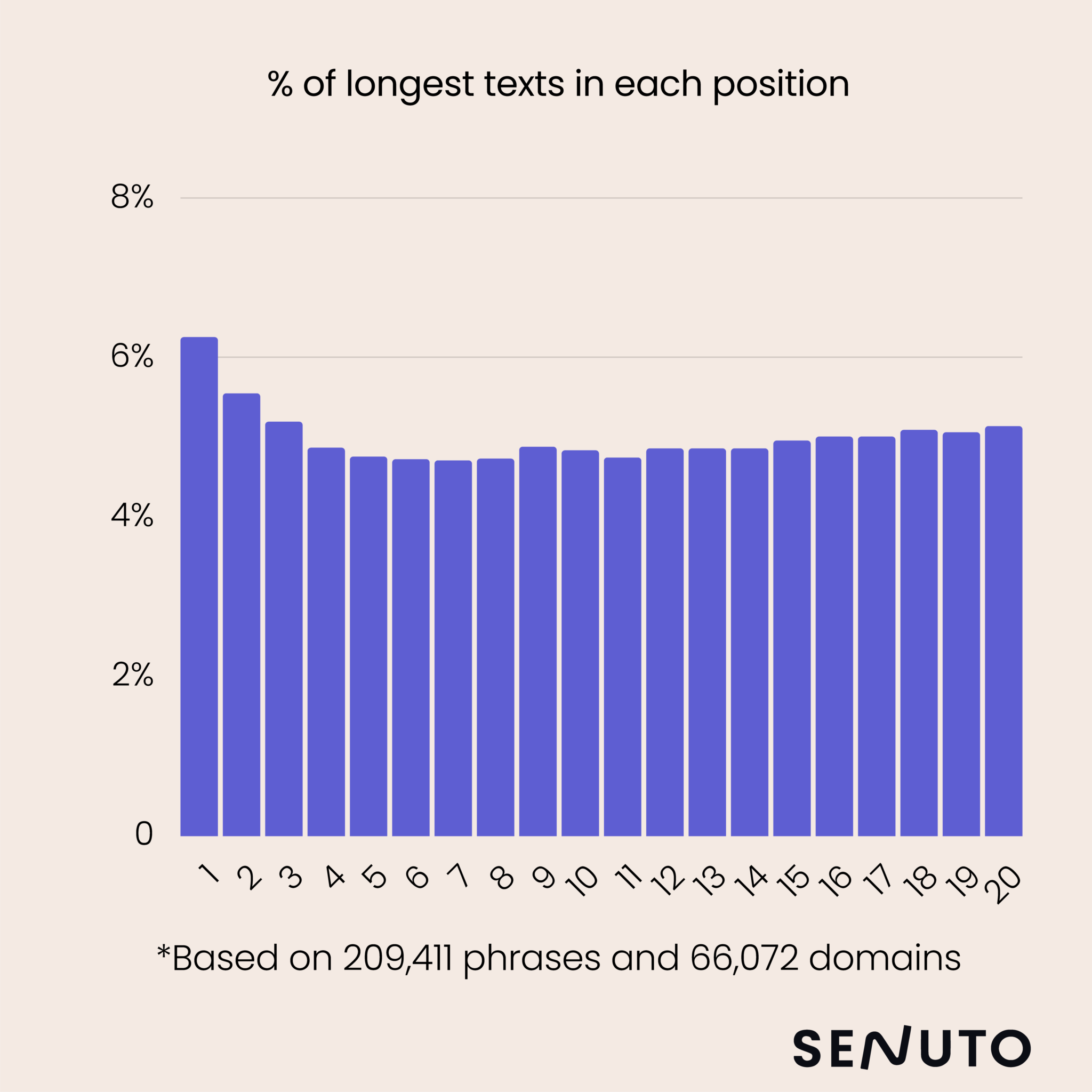
When examining the correlation of content length with position, we get a result of 0.08.
It is therefore not worth focusing your attention on the length of the content. The overriding goal should be to satisfy the user’s intent. The content should cover all the concepts, entities and contexts in a given set of concepts in the simplest and shortest possible form.
This is where the question may arise: what then of the guidelines for copywriters in terms of article length?
I recommend that the length of the content in a copywriter’s brief should be a recommendation, a certain benchmark, rather than a rigid requirement. In this respect, the guidelines provided by the Content Planner tool in Senuto are very useful.
The content length aspect can be disregarded in specific situations – when the copywriter is very experienced and understands SEO to the extent described here. However, copywriters with SEO knowledge at this level are likely to be few in number, so recommendations on content length will still be a valuable guideline.
2. Google does not understand Polish
I regularly hear this statement.
Google has NLP models capable of processing Polish (such as BERT). They may not be as well-trained for Polish as for English, but they work with high efficiency. Let the Allegro-trained BERT model, which I described in the chapter on the history of Google’s algorithm development, serve as an example.
Sometimes, of course, an error will occur in the search results, but an isolated incident should not form the basis for such conclusions.
Another example is the GPT-3 model, with which we can, among other things, automatically generate content. It handles the Polish language very well. Google has similar and even better models.
3. LSI keywords have a significant impact on semantic SEO
In many articles on topical authority, we find that this issue is linked to LSI (latent semantic indexing) keywords – those words and phrases that are semantically related to the main topic of the content.
If the content is about weightlifting, then according to this theory we should also use words such as gym, weight loss, personal trainer.
John Muller himself emphasised in one of his tweets that LSI keywords do not exist:
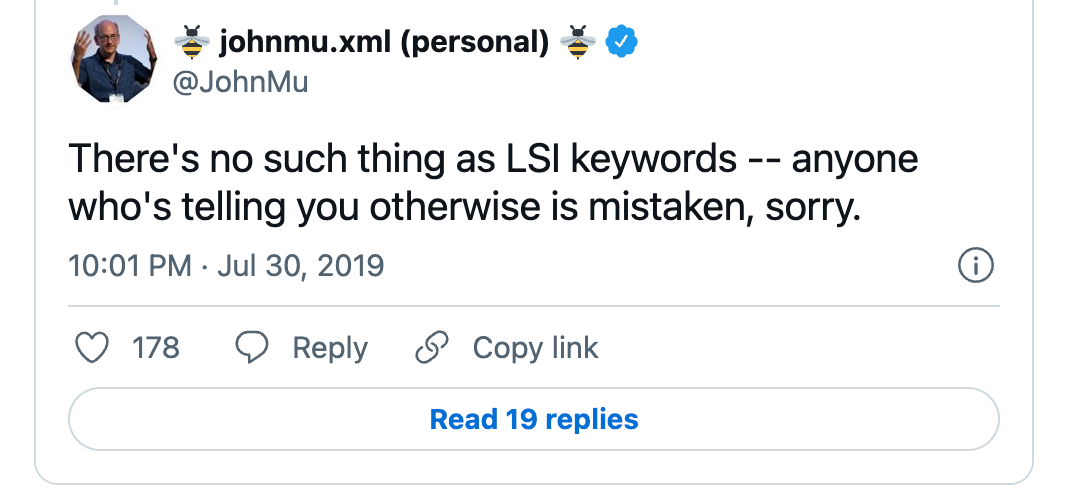
Latent semantic indexing is a method that dates back to 1980 (more than 40 years!). It is a simple NLP technique to explore the relationship of words to the documents containing those words.
Google with technologies such as neural matching or RankBrain would not have used such an old technology.
Many people who suggest using LSI keywords confuse them with Google’s use of synonyms and semantically related words. These are completely two different processes.
So don’t use tools to determine LSI keywords and their recommendations.
How to put semantic SEO techniques into practice?
You are already quite familiar with the concepts of semantic SEO, in this part of the article we will look at the practice.
Below, you will find a list of tips on how to incorporate this theoretical knowledge into your daily work.
1. Change your thinking about keywords
I mentioned that with Rank Brain you don’t have to focus so much on the long tail. Google groups phrases that meet the same intent together. This allows you to appear high for phrases you don’t even have in your content.
Your goal should be two things:
- Ontology: describing concepts with as many attributes as possible in as simple a form as possible.
- Taxonomy: the appropriate arrangement of these in the service structure.
Of course, don’t abandon keywords altogether. Google still analyses pages from this angle. However, you do not have to use both “what to do in Warsaw” and “attractions of Warsaw” in your text.
Google is first and foremost supposed to understand the content and structure of a page. If your page appears in a direct answer snippet, it may mean that Google is coping with its analysis.
2. Maintain a logical structure in the content
In the guidelines for the quality team, we can read that Google divides content into 3 parts:
- Main content
- Ads
- Supplementary Content
Google considers the more relevant content to be that found in the section “above the fold” (before scrolling). Therefore, starting with the title tag, it is advisable to place the most important information at the top, and the less important and more detailed information below.
Among other things, Google has patents on content header analysis. Therefore, all content should be very logically composed:
- The most important content should be conveyed in the topmost headings.
- Each headline and the content underneath it should convey a unique message. If two headlines are on the same topic – combine them.
3. Fill in the knowledge gaps
Try to provide unique knowledge on the topic, one that the user will not find in the TOP10 search results. Don’t just try to duplicate what is already in the TOP10 search results.
Don’t just focus on keywords that have a high number of searches. Those with very few or no searches are also worth responding to. Therefore, don’t exclude phrases that, according to the Senuto Keyword Database, have, for example, 0 or 10 searches per month – you may not catch traffic from Google with them (at least for now!), but they will influence Google’s evaluation of your content.
4. Use the schema.org dictionary to describe your site
Although the use of schema.org does not directly affect search engine rankings, it allows the search engine to better understand the structure of your site – and is therefore beneficial to you.
5. Publish regularly and refresh content
Regular publishing will increase your update score, which will translate into a better crawl budget. Your content will be indexed faster.
Refreshing content whose intention has changed is equally important.
6. Look broadly at the intention
Try to match the type of content to the user’s expectations.
If mainly short content appears in search results, don’t write a long article out of spite. If lists or tables appear in the search results, try adding them to your content. User intent is not just about the type of sub-page you want to display to the user. You need to approach the topic in much more detail, deepening your understanding of what user needs are being met by the TOP10 Google results. From these results, we are able to decipher whether the user’s intention is long text or table-formatted content, or perhaps something else.
7. Don’t look at the length of the content
The length of content does not matter in SEO. Content should be as short as possible. SEO is not a race for content length, it’s about satisfying user intent. Again: analyse the results from the TOP10 of Google. If you see that the user primarily expects long content – write such an article, if he or she expects concise information – provide that.
Act on logic: if a user types in a general query (such as “SEO”), this most likely means that they are looking for general information and you need to give them more knowledge than you would for a specific query (such as “how robots.txt affects SEO”). Text answering a general query will generally be longer.
Keep in mind that in some cases, too much content can adversely affect your position.
8. Use short sentences and phrases
NLP models are much better at understanding short sentences (you can test this in the Google NLP API under the ‘dependency tree’ option). This way of writing is also generally better absorbed and preferred by users.
9. Be careful about expressing your own opinions in content
Google, with the help of machine learning models, tries to recognise what in content is opinion and what is fact (using entity dictionaries, among other things). Typically, Google is less keen to show the opinion of content authors in search results, so instead of basing your opinions – focus on facts.
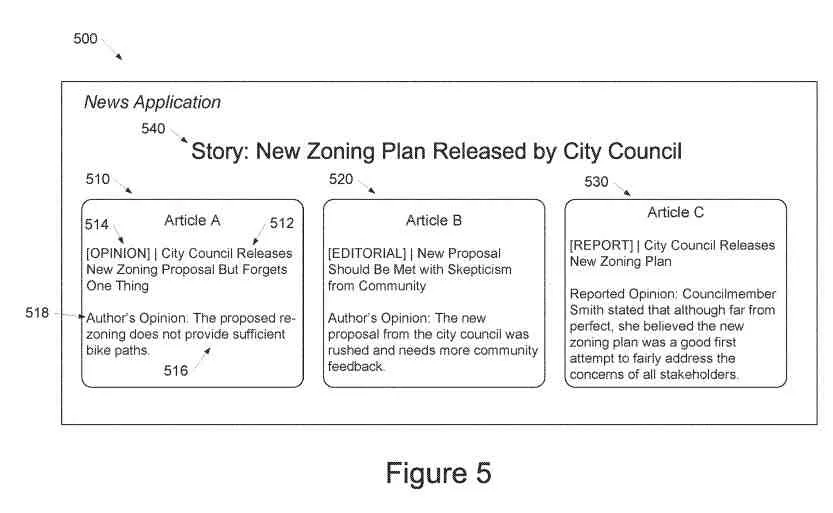
You can read more about this on the SEO by the sea website.
10. Exercise patience
Every SEO specialist is taught to be patient. Semantic SEO is no different.
- We publish content regularly, increasing over time
- Building an internal link network
In some cases, building a high topical authority may require writing several hundred articles. Additionally, the search engine needs time to understand the structure of the site and classify it as a valuable source of information. A few months is a good time to start expecting results.
How will Senuto help you with semantic SEO?
We already had a content planning service on offer since 2016, in line with semantic SEO principles. We want to develop our app in this direction as well. One module that can support the building of strong topical authority is our Senuto Content Planner. In this part of the article, we will see how this tool addresses the issues described in the article.
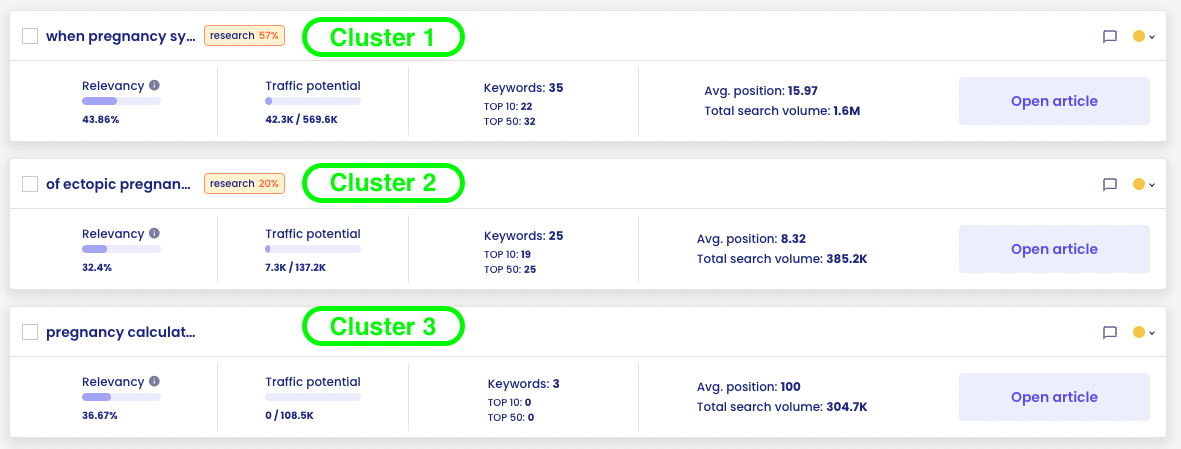
In the chart above, there are three example topic clusters from the area of pregnancy. For each of these, Content Planner determines:
- Potential movement
- Number of keywords assigned to the cluster
- Current visibility of the service within the cluster
A single cluster represents a single article or sub-page that needs to be created (or the current one optimised) on the site. The keywords assigned to a cluster are those for which the target subpage created should appear in search results.
Clusters are also combined into higher-level groups, using graph techniques. These groups are designed to map the placement of individual clusters next to each other in the site’s information architecture (remember the bit of text about pillar pages and lower and higher level clusters?). It is also useful to add internal links between clusters in one group.
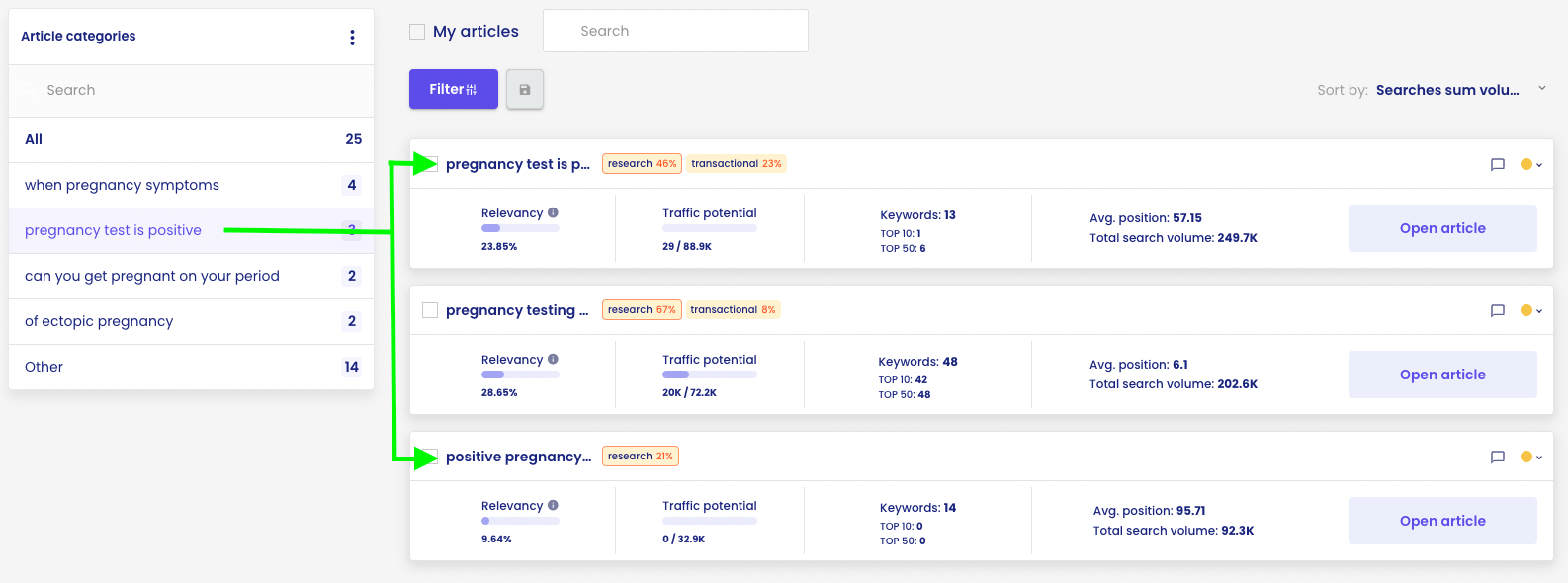
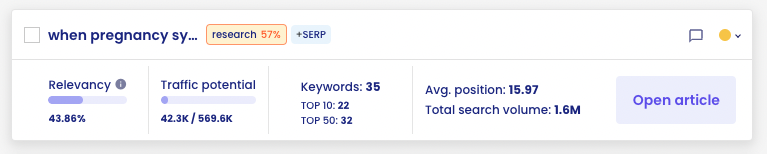
E.g. the ‘when pregnancy symptoms’ cluster has primarily a research intention (dominant interpretation, 57%).
For an even better understanding of the user’s intentions, the system carries out an analysis of the search results – you can see exactly what is in these results for the keywords assigned to the cluster.
The first important element is the snippets that appear in the search results.
For the ‘pregnancy symptoms’ cluster, the dominant snippet is direct answer (occurs on 6 out of 7 keywords).
The second important element is the competitive analysis.
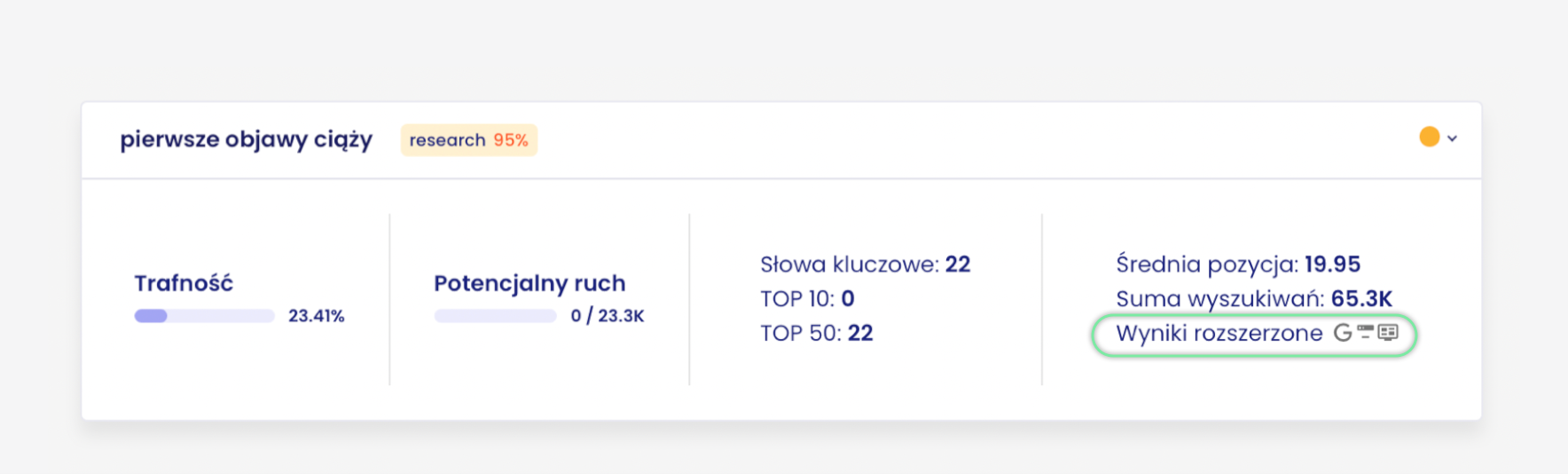
For the cluster ‘first symptoms of pregnancy’, the dominant snippet is direct answer (occurs on 14 out of 22 keywords).
The second important element is the competitive analysis.
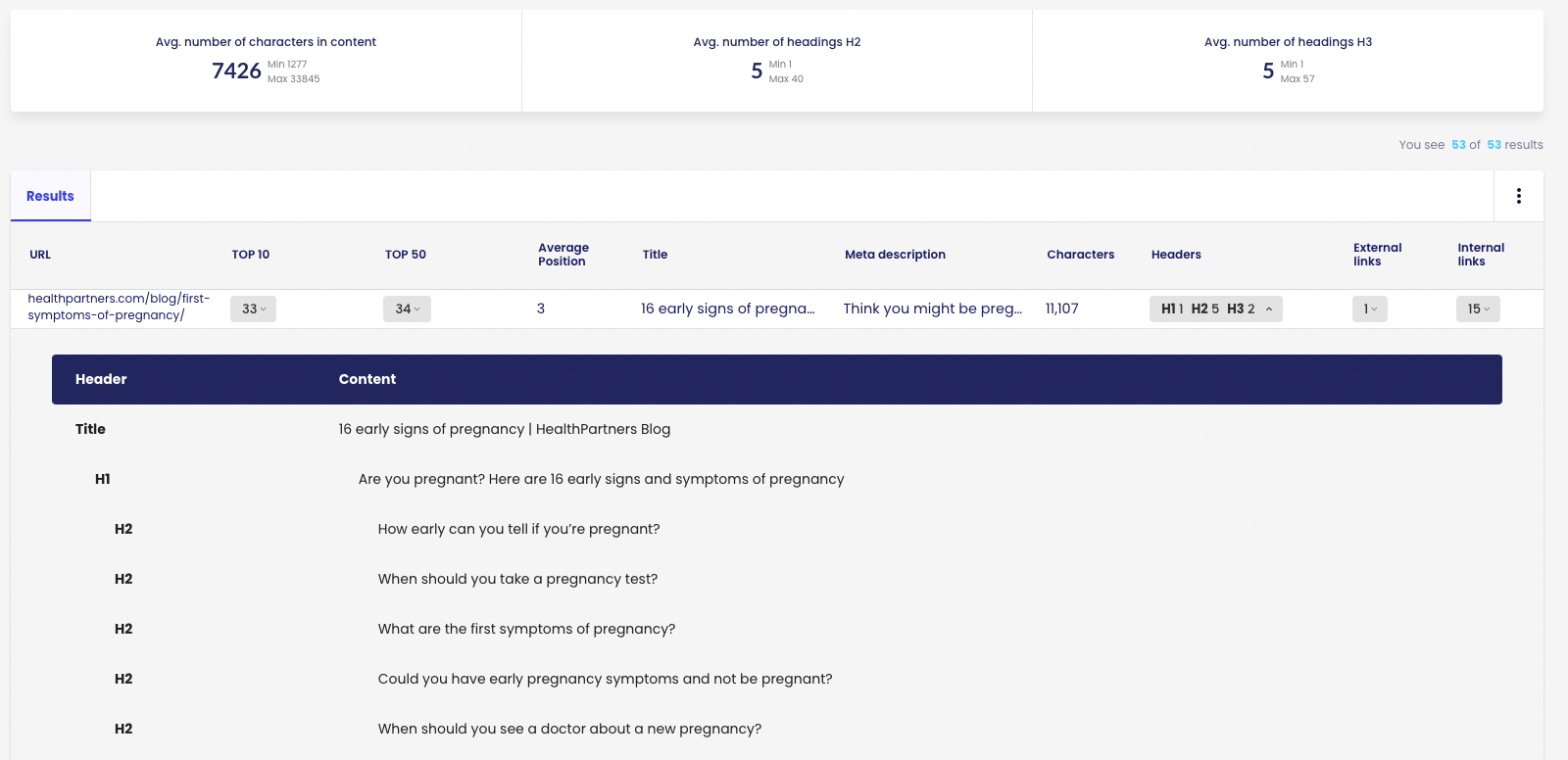
The system analyses parameters such as:
- Average length of competition content
- Number of headings
- Number of internal links
- Meta data
- Structure of each competitor’s headlines
Such analysis is a set of important clues as to how to respond to the user’s intention with their content.
In addition, the system suggests which user questions you should answer in your content. From reading this article, you know how important this is.
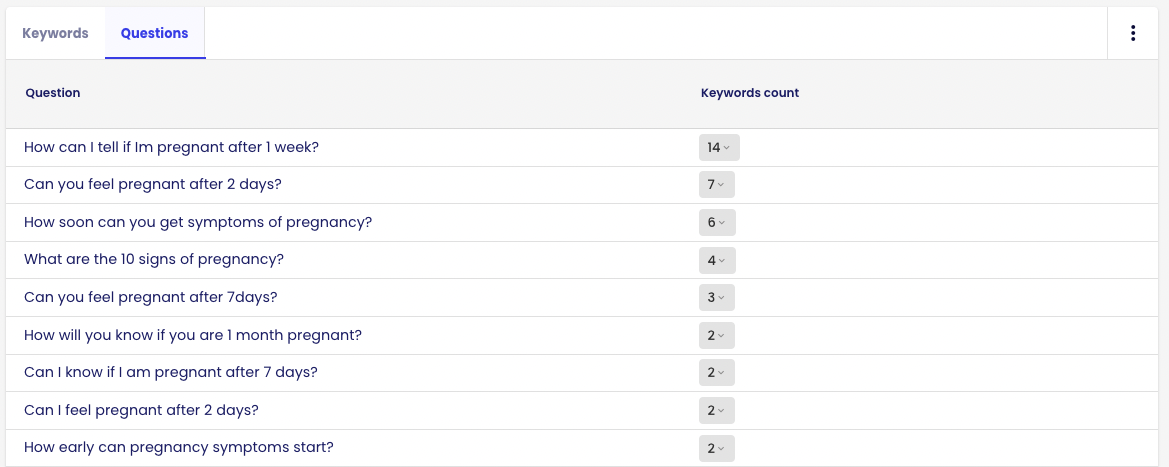
If you want to perform clustering on a customised keyword database, you can use the Keyword Clustering tool in Senuto.
If you are not yet using Senuto’s Content Planner, be sure to try it out along with the second, complementary Content Writer module.
Both of these modules are milestones for us in developing a tool that will allow you to take full control of your semantic SEO process.
The future of semantic SEO
Looking historically at the development of the search engine, it is not difficult to predict that its future will be very interesting. Google will certainly develop more NLP and other machine learning models to understand user intent and respond to it even better. We will see changes over the coming years. The growth in AI capabilities is growing exponentially.
At the moment, I find one change that is likely to mess up the search results a lot in the near future.
Multitask Unified Model (MUM)
Google has already announced another major change in the way it analyses information and presents it to the user in May 2021 – the introduction of the MUM, Multitask Unified Model, to the search engine. Multitask is the key word. It is not an NLP model like BERT, which is used for language processing. MUM can process and understand different types of content (text, images, video, audio). According to Google’s claims, it is 1000 (!) times more effective than BERT.
The graphics below show how the MUM will work:
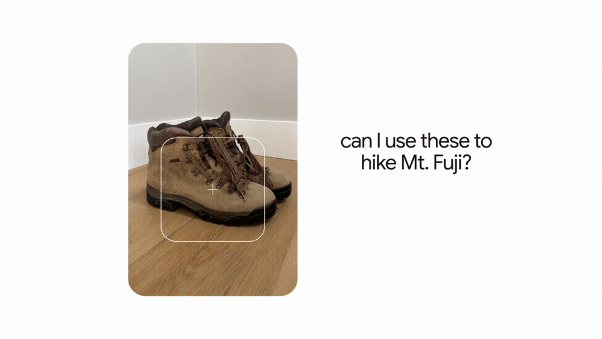
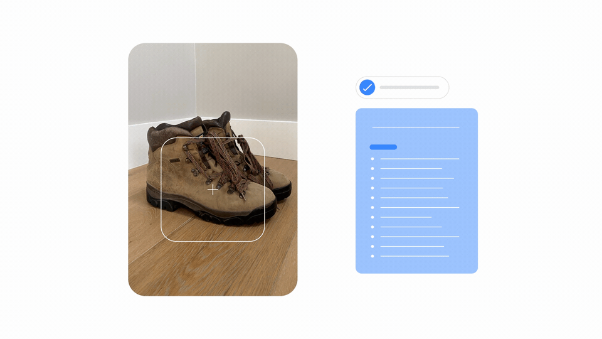
…and answer
Mum will be able to combine a photo with an enquiry and provide comprehensive information, in this case: can I climb Mount Fuji in these shoes?
MUM runs on the T5 (Text-To-Text Transfer Transformer) model that Google published in 2020. It is an open-source model (just like BERT) – which means that if you want to try it out, you can use the model prepared by Google Colab engineers.
As with BERT, T5 fine-tuning can be carried out for a variety of tasks.
The complexity of the T5 model is shown in the graphic below:
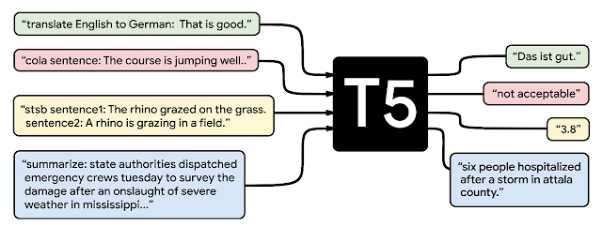
źródło: https://ai.googleblog.com/2020/02/exploring-transfer-learning-with-t5.html
The aim of this change is to provide the user with information in fewer queries than before. The principles described above will be even more important.
Google also uses its proprietary PaLM language model, which is trained on 540 billion parameters.
In comparison, the GPT-3 model, which has recently been highly popularised by various automatic content generation tools, has been trained on 175 billion parameters.
The graphic below shows what the model is capable of depending on the number of parameters:
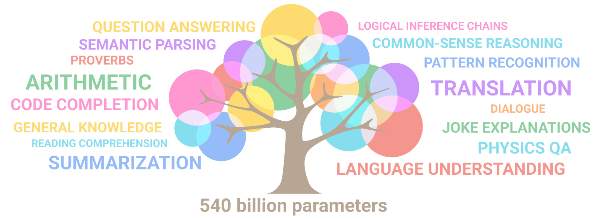
źródło: https://ai.googleblog.com/2022/04/pathways-language-model-palm-scaling-to.html
In a study in which AI models are tested on 200 different tasks (a list of which can be found here), PaLM performs better than the average human.
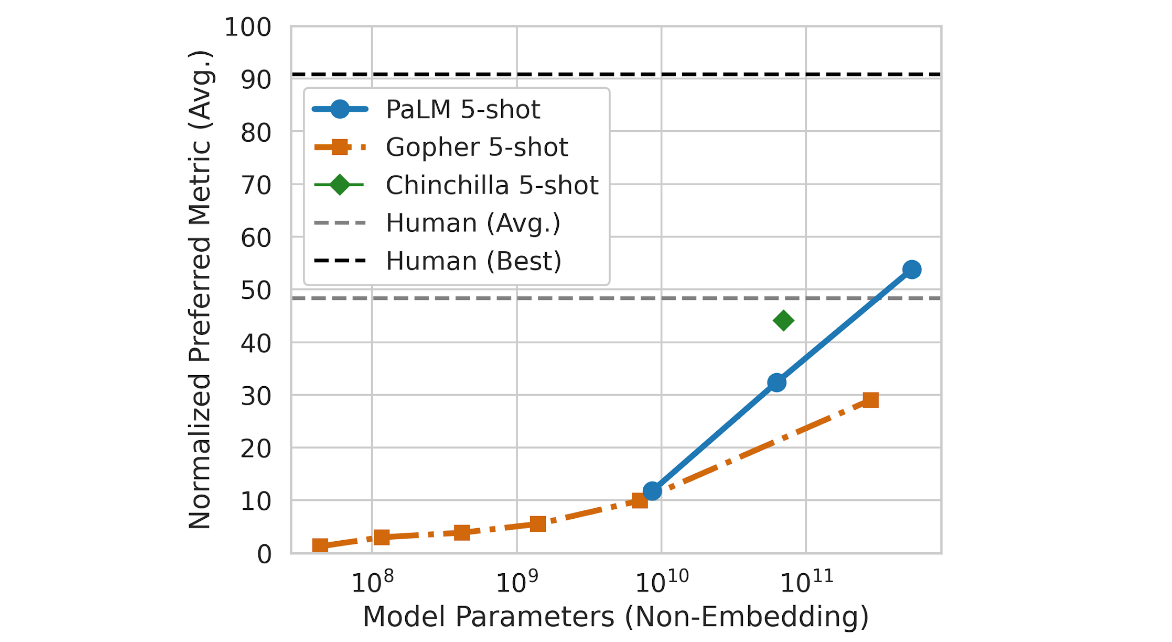
źródło: https://ai.googleblog.com/2022/04/pathways-language-model-palm-scaling-to.html
It also performs much better than other popular models (including the GPT-3):
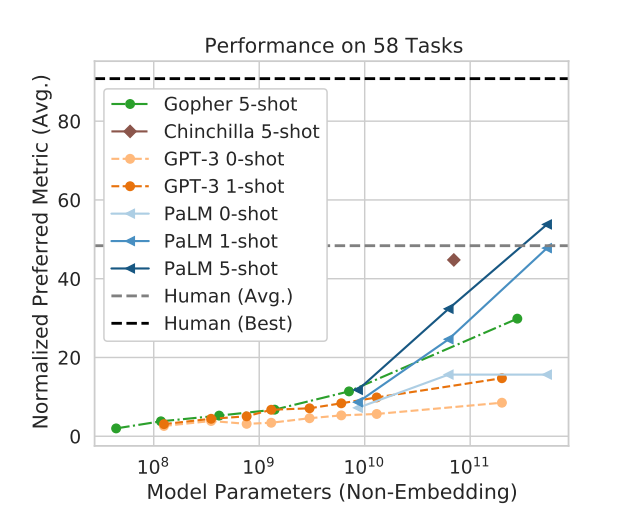
źródło: https://arxiv.org/pdf/2204.02311.pdf
One good example is the ability to translate jokes.
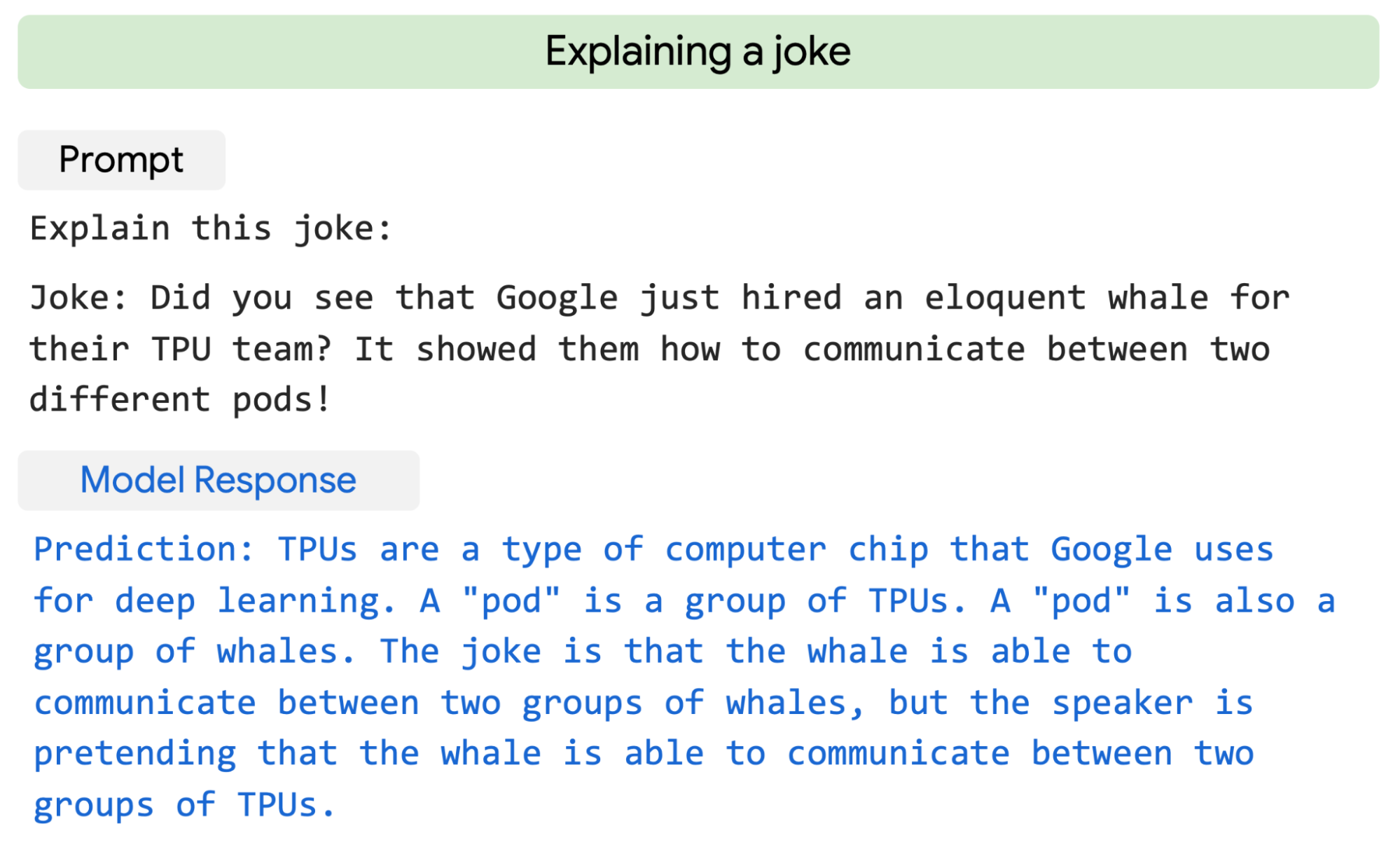
źródło: https://ai.googleblog.com/2022/04/pathways-language-model-palm-scaling-to.html
You can read more about PaLM on Google’s AI blog and here (pdf).
Google’s AI blog is worth following, as they keep you up to date with their new developments in artificial intelligence. Reading this blog gives a good insight into what resources Google has at its disposal.
The number of models and their capabilities are growing at a very fast pace. Already the GPT-4 model, which is expected to be available in a few years, is said to be trained on 100 trillion parameters (what will it be capable of?).
If Google is able to generate quality content on a large scale, then perhaps in future simple questions will be answered by AI-generated content rather than a list of websites.
However, models are always trained on some basis (e.g. GPT-3 ceased learning in 2019), so if you provide new and unique knowledge according to the principles described in this article, you will remain in the search engines’ focus area.
Completion
Thank you for reading. I hope that the concepts of semantic SEO are clear to you and that you will start to put this knowledge into practice. For my part, I can assure you that we will update you on our blog and provide you with the latest knowledge in this area.